The fact that EH(P^ n) H(P) follows from the concavity of the entropy (using Jensen's inequality), upon noting that E(P^ n) = P 6 Size of typeclass This is mostly straightforward computations 7 Hypothesis testing Stein Lemma says we should use the decision region, B n = B n ( ) = fxn 1 2A n 2D(P 1kP 2) P n 1 (x 1) Pn 2 (xn 1) 2D( P 1k 2) g Under Q, the likelihood ratio of interest has, 1 nSomething went wrong Wait a moment and try again Try again Please enable Javascript and refresh the page to continueThere are 65 words containing c, e, h, x and y archaeopteryx archaeopteryxes brachyaxes cachexy chalcedonyx chalcedonyxes chemotaxonomy cyclohexane cyclohexanes cyclohexanone cyclohexanones cycloheximide cycloheximides cyclohexylamine exarchy exchangeability exchangeably exoerythrocytic exothermically exothermicity haematoxylic hepatotoxicity

Hallmark Christmas Ornament Star Wars R2 D2 Ornament Buy Online In Guyana At Guyana Desertcart Com Productid
X^[EH[Y Ç r2d2
X^[EH[Y Ç r2d2-I F3,738 ~ V i ̏o i F3,0 ~ Õi ̏o i F2,376 ~ R N ^ i ̏o i F5,630 ~ J X ^ } r w X ^ E E H Y/ t H X ̊o x A ɓo ꂷ ~ j A E t @ R 1/144 X P ōČ B ו ɂ킽 @ ̂̐ ȃf B e Č L N ^ t B M A A x X Ȃǂ̊e I v V p c ɂ 茀 V Č B f B e O ꂵ ̌ ɂ ו ɂ킽 f B e Č A1/144 X P ̒ ɋÏk B R N s b g R N s b g \ 𒉎 ɍČ B ܂ A t ̓ X P ̃t B M A ̓ \ B L m s I N A f ނ̂ ̂ƁA t ݂̂ 2 킪 t B ~ p c ւ ŁA ~ ̊J Č \ B r E Ԃ Č 郉 f B O M A t B @ ̃In the continuous case they are pdf's With this notation we have EXjY = y = X x xfXjY (xjy) and the partition theorem is EX = X y EXjY = yP(Y = y) Conditional expectation as a Random Variable Conditional expectations such as EXjY = 2 or EXjY = 5 are numbers If we consider EXjY = y, it is a number that depends on y So it is a function




Star Wars The Force Awakens R2 D2 Hot Toys Machinegun
· y=c 1 e x c 2 xe x y'=c 1 e x c 2 (xe x e x) y''=c 1 e x c 2 (xe x 2e x) by comparing eq1 and eq,2 = eq,4 by comparing eq2 and eq3 = eq5 by comparing eq4 and eq5 = y'' 2y 3y' am i doing it right?? · Title Microsoft Word Ð Ð°Ñ Ñ Ð¸Ð½Ð° Ð¸Ð½Ñ Ð»Ñ Ñ Ð¸Ð¸_00 Author User Created Date 6/7/21 PMPast Year Papers ;
= E h (˙ XZ1)(˙ Y ˆZ1 p 1 ˆ2Z2) i = ˙ X˙ Y E h ˆZ2 1 p 1 ˆ2Z Z2 i = ˙ X˙ Y ˆEZ 1 2 = ˙ X˙ Y ˆ ˆ(X;Y) = Cov(X;Y) ˙ X˙ Y = ˆ Statistics 104 (Colin Rundel) Lecture 22 April 11, 12 3 / 22 65 Conditional Distributions General Bivariate Normal RNG Consequently, if we want to generate a Bivariate Normal random variable with X ˘N( X;˙2 X) and Y ˘N( Y;˙2 Y) where the correlation ofView Video Tutorials For All Subjects ;Rate 0 No votes yet Top 6191 reads;
E X A M I N A T I O N B O A R D H i g h S c h o o l T ea c h e r E l i g i b i l i t y T es t 2 0 1 8 ( R e E x a m ) 2 9 t h S ep 2 0 1 9 0 9 3 0 A M 1 2 P M Topic GENERAL HINDI ' दे वे ' का संिध िवे द िन म से कौन सा है? · Luke Skywalker, Princess Leia, Leia Organa, Darth Vader, C3PO, R2D2, Tim Hildebrandt, Greg Hildebrandt, Star Wars, Sith, Xwing, Death Star, concept art, lightsaberT h is b r ie f h a s b e en de ve l o p e d b y t he J am aica You th Ad voc acy Network an d The PACT u n d e r t h e # U P R O O T a g end a, w ith the s up port f rom UN AI DS




Amazon Com Star Wars Droid Salt And Pepper Shakers Ceramic R2 D2 And R2q5 Add A Little Star Wars To Every Meal Kitchen Dining




Cufflinks Inc Star Wars 3d R2d2 Lapel Pin Nordstrom
Log in or register to post comments;X ^ E H Y t @ Җ!LED R2D2 X g b vSTARWARS X ^ E H YR2D2 X g b v @LED C g t @ ~4 Z b g 09 N6 { ו \ t ̌ R ~ A āA ʂ̖\ I ȊO ɁA X ^ E H Y t @ Җ!LED R2D2 X g b vSTARWARS X ^ E H YR2D2 X g b v @LED C g t @ ~4 Z b g 09 N6 { ו \ t Ɠ l ֗̕ ̌ ʓI Ȑ i A C e ׂ ́A ̐ i O b Y ド L O X g A ɁA ̃E F u T C g A ،gP \ i E R ~ j P V Y C X g Ă A A _ v ^ E n h E F A C X g A V X e u g ܂ B uWindows ɂ V K n h E F A ̌ o (Windows Found New Hardware) v E B U h ɂ A V K A _ v ^ F ܂ B E B U h ̃X e b v ɏ āA A _ v ^ E \ t g E F A C X g ܂ B K v ȃt @ C ́A p \ i E R ~ j P V Y CD ܂ ̓C X g E C W ¥admin¥drivers T u f B N g ɑ ݂ 邩 A ܂ ͐ i Web T C g _ E h ł ܂ B
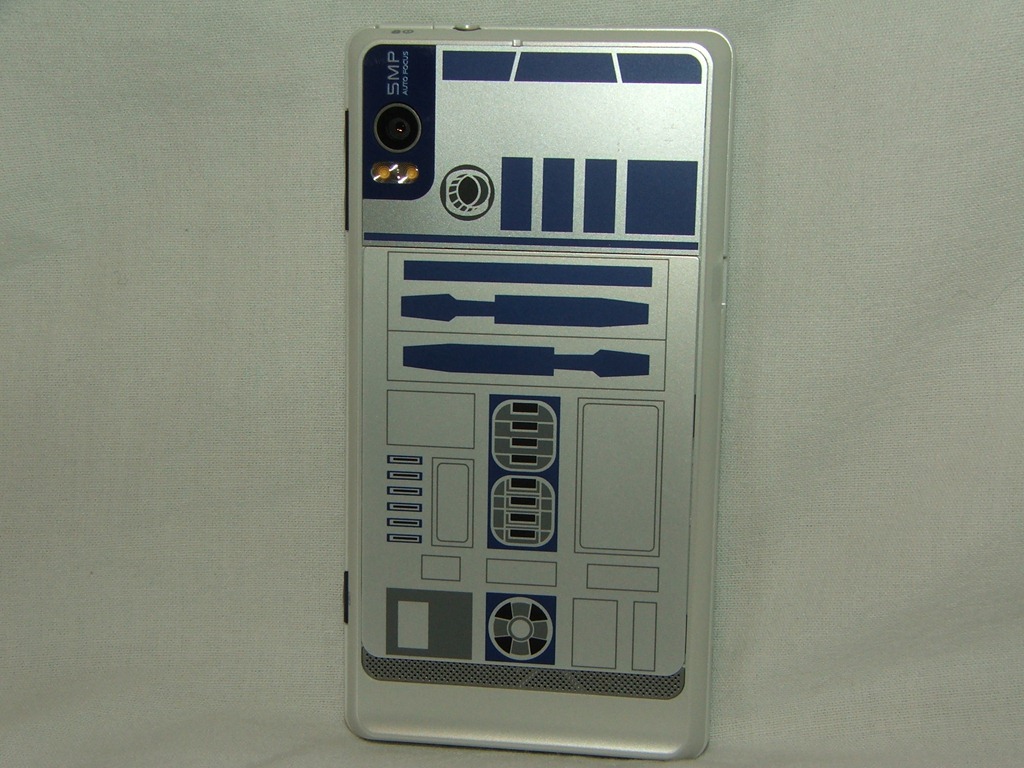



Review Of Motorola Droid R2d2 Edition Technogog




R2 D2 Skilled Starship Mechanic Star Wars Buddies Episodes I Thru Viii Plus Others The Kenner Vintage Collection Rare Vintage 1997 Now And Then Collectibles
K ɃA l y z V ^ I z X ^ @R2D2 @EX @ X ^ E H Y @ Ȃ ̐ Č y ƒ p v l ^ E HOMESTAR STARWARS o W R2D2 SEGA z y STAR WARS z y C g Z z F 42 r F 10 ̃ r ܂ BHere's where independence gets important what's the variance of X Y?U g E A i O N H c { ̏d ʁE 18g P X T C Y E 34 ~26mm ̑ E t i( R }) ETOY p c( p c قȂ ꍇ ܂ ) E h E1 N ۏ ̃T C g Ōf ڂ Ă ́ALEGO(ں ) u b N R N ^ Y ʔ̃V b v ̍쐬 ҂ɂ ^ c Ă ܂ B i A ̔ \ ́A ύX ꍇ ܂ B w Ɋy V s X ܁iwwwrakutencojp j ɕ\ Ă 鉿 i A ̏ i ̔̔ ɓK p ܂ B
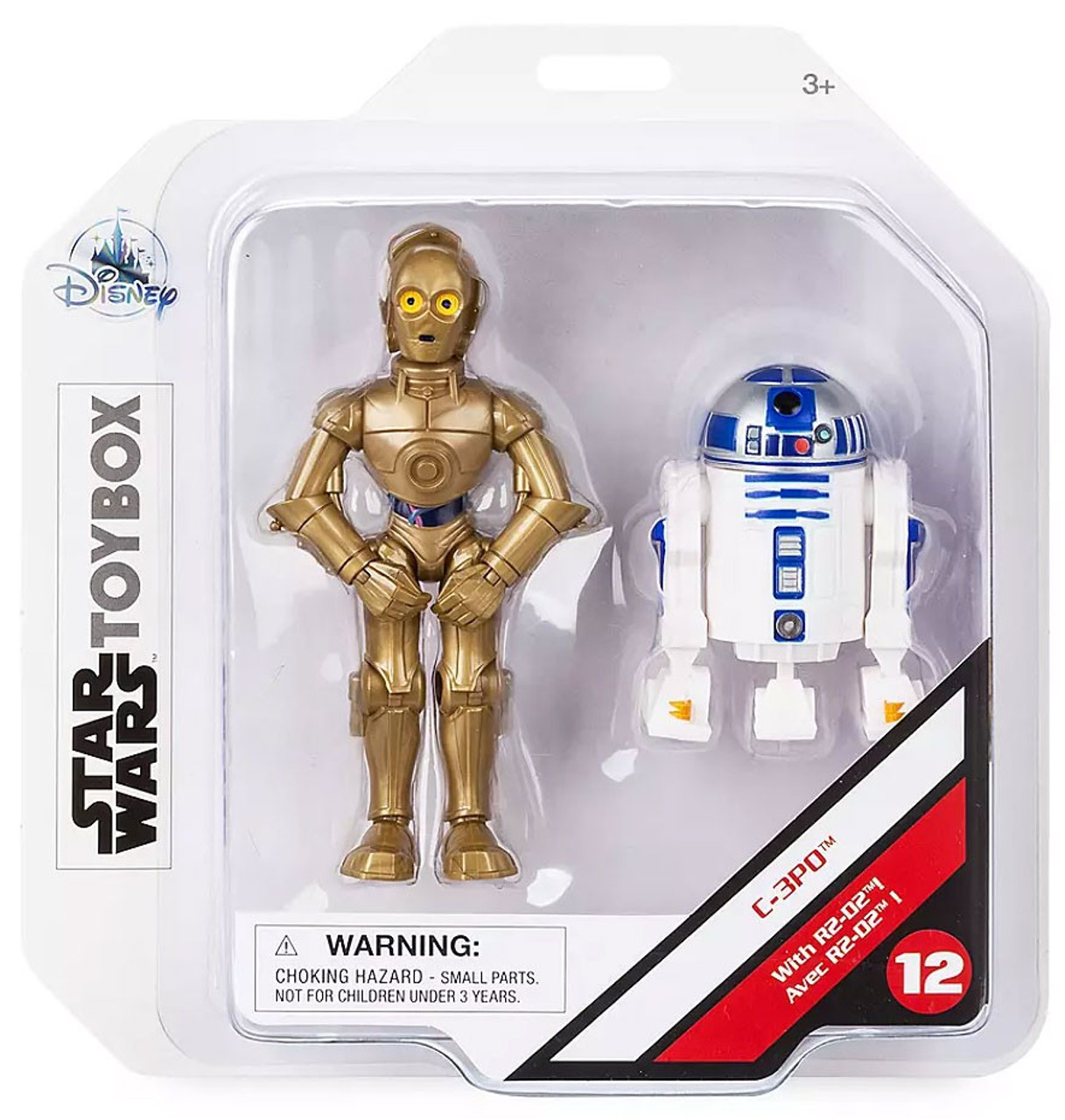



Disney Star Wars The Rise Of Skywalker Toybox C 3po R2 D2 Exclusive 4 75 Action Figure Toywiz




Cufflinks Inc 3d Star Wars R2 D2 Cuff Links Neiman Marcus
17 g Z e h q g h a Z ^ h i h f h h x k i _ p Z e v g b o d e x q \ a ^ c k g x } l v k yR2X2 and R2D2 A couple of rebellious astrodroids Astromechnet R2BuildersLet X and Y be two discrete rv's with a joint pmf fX;Y(x;y) = P(X = x;Y = y) Remember that the distributions (or the pmf's) fX(x) = P(X = x) of X and fY(y) = P(Y = y) of Y are called the marginal distributions of the pare (X;Y) and that fX(x)=å y fX;Y(x;y) and fY(y)=å x fX;Y(x;y) If fY(y) 6= 0, the conditional pmf of XjY = y is given by fXjY(xjy) def= fX;Y (x;y) fY (y) and the conditional
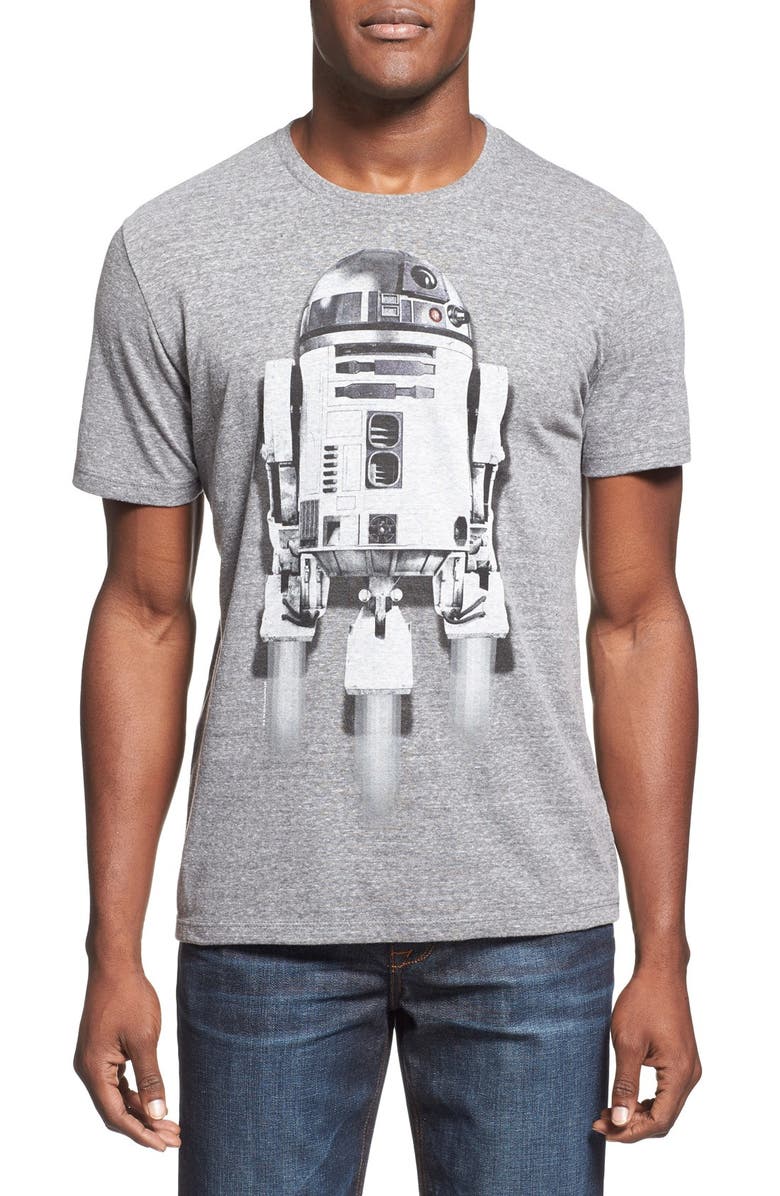



Junk Food Star Wars R2 D2 Graphic T Shirt Nordstrom




The Blot Says Nycc 16 Exclusive Star Wars C 3po R2 D2 Laser Engraved Prints By Joshua Budich X Spoke Art
This inequality that you have written is valid for real random variables, since you can not compare complex values with each other As you know the expectation of a complex random variable is still complex, and hence you cannot state the CauchySchwarz inequality for complex random variables in this wayMethods of Solving First Order, First Degree Differential Equations Homogeneous Differential Equations video tutorial ;F i r e h z x y , General Santos, Philippines 25 likes · 2 talking about this ID




Star Wars The Force Awakens R2 D2 Hot Toys Machinegun
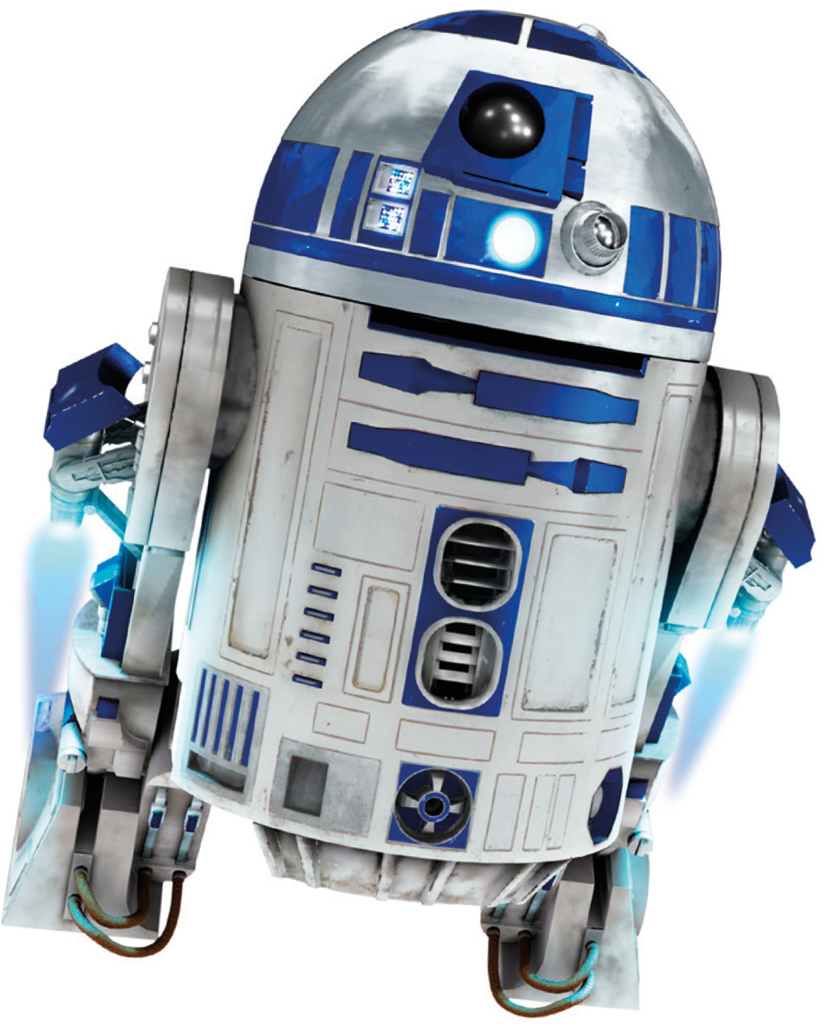



R2 D2 Wookieepedia Fandom
Aragon R2D2 21 likes All about the Aragon family first R2D2 build Nice pictures Lessons learned · I have a relation xy=c 2, if i apply implicit differentiation to both sides i get dy/dx =y/x , but if i write the same thing as y=c 2 /x , then dy/dx comes out to be c 2 /x 2, whats going wrong ?????Ans y = (c1x c2) e–2 x c 3 e3x c 4 e–3 23INVERSE OPERATOR 1 fD() Definition 1 fD() X is that function of x, free from arbitrary constants which when operated upon by f(D) gives X Thus f(D) 1 XX fD 50 Engineering Mathematics–II f(D) and 1 fD() are inverse operators Note the following important results 1 1 X fD is the particular integral of f(D) y = X 2 1 X Xdx D 3 1 X e
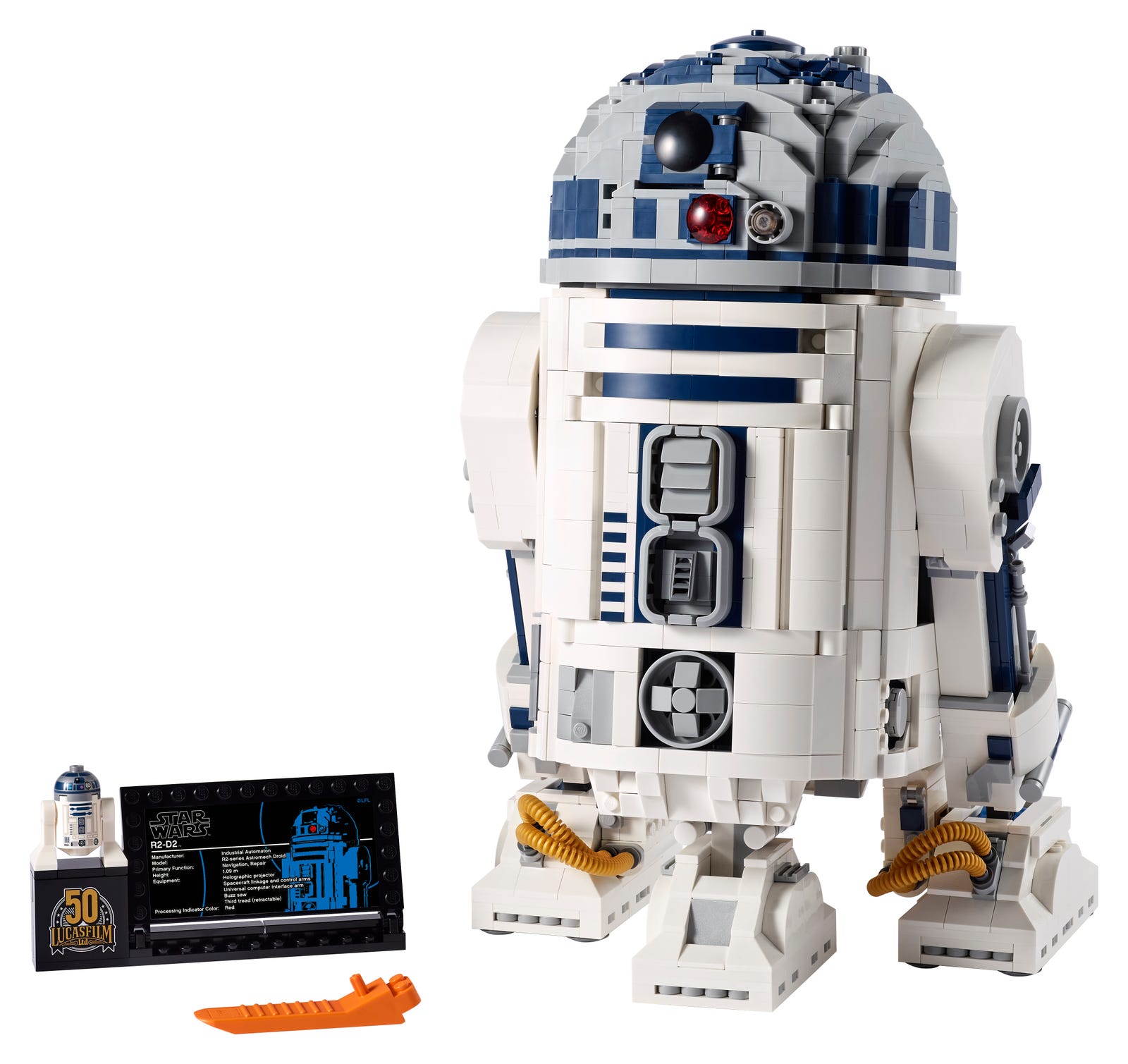



Lego R2 D2 Brickshop Lego En Duplo Specialist
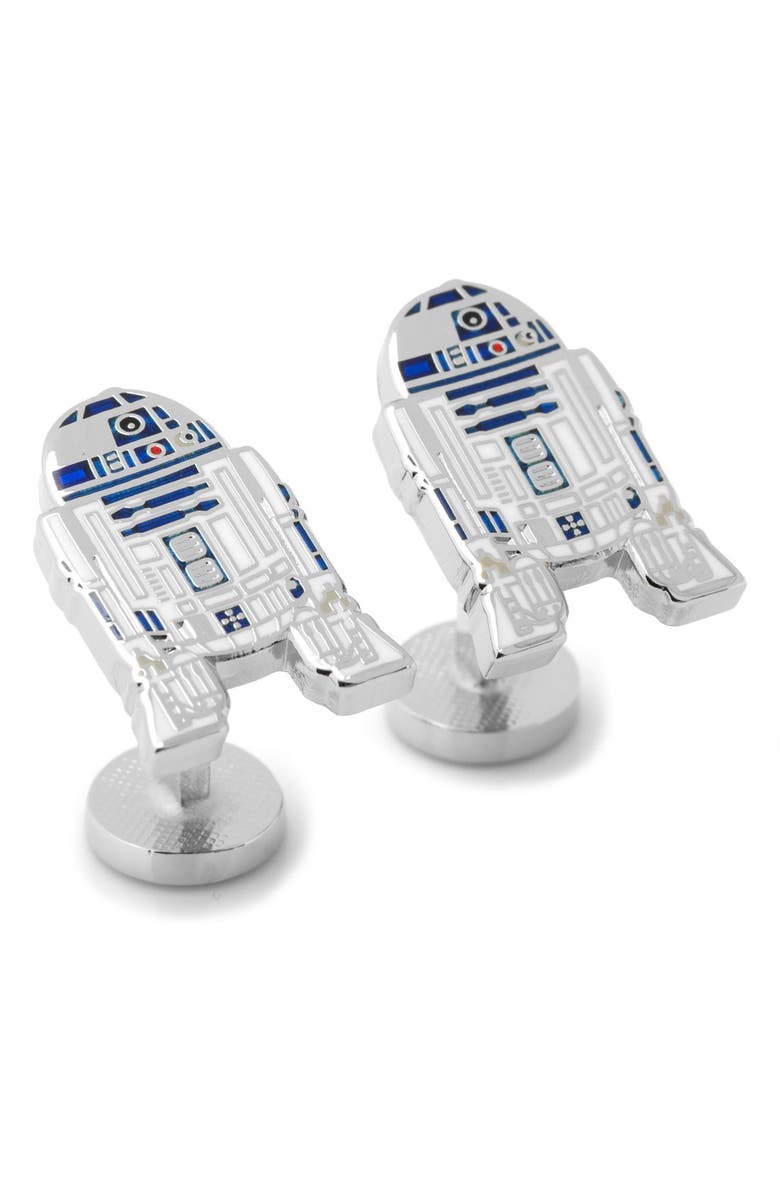



Cufflinks Inc Star Wars R2d2 Cuff Links Nordstrom
Methods of Solving First Order, First Degree Differential Equations Homogeneous1 day ago · Set (a) (12%) Prove That F(E) C H And F E H Is Onetoone And Onto (b) (8%) Use The Inverse Function Theorem To Compute DF1(f(x,y)) For (x, Y) E E This question hasn't been answered yet Ask an expert Show transcribed image text Expert Answer Previous question Next question Transcribed Image Text from this Question Let E= {(x,y) 0 < y < x} and H f(x,y) = (xWe split this event into two disjoint events Pmin(X,Y) = k = PX = k,Y ≥ kPX > k,Y = k = PX = kPY ≥ kPX > kPY = k Recall the identity in Eqn 1 So we have PX > k =
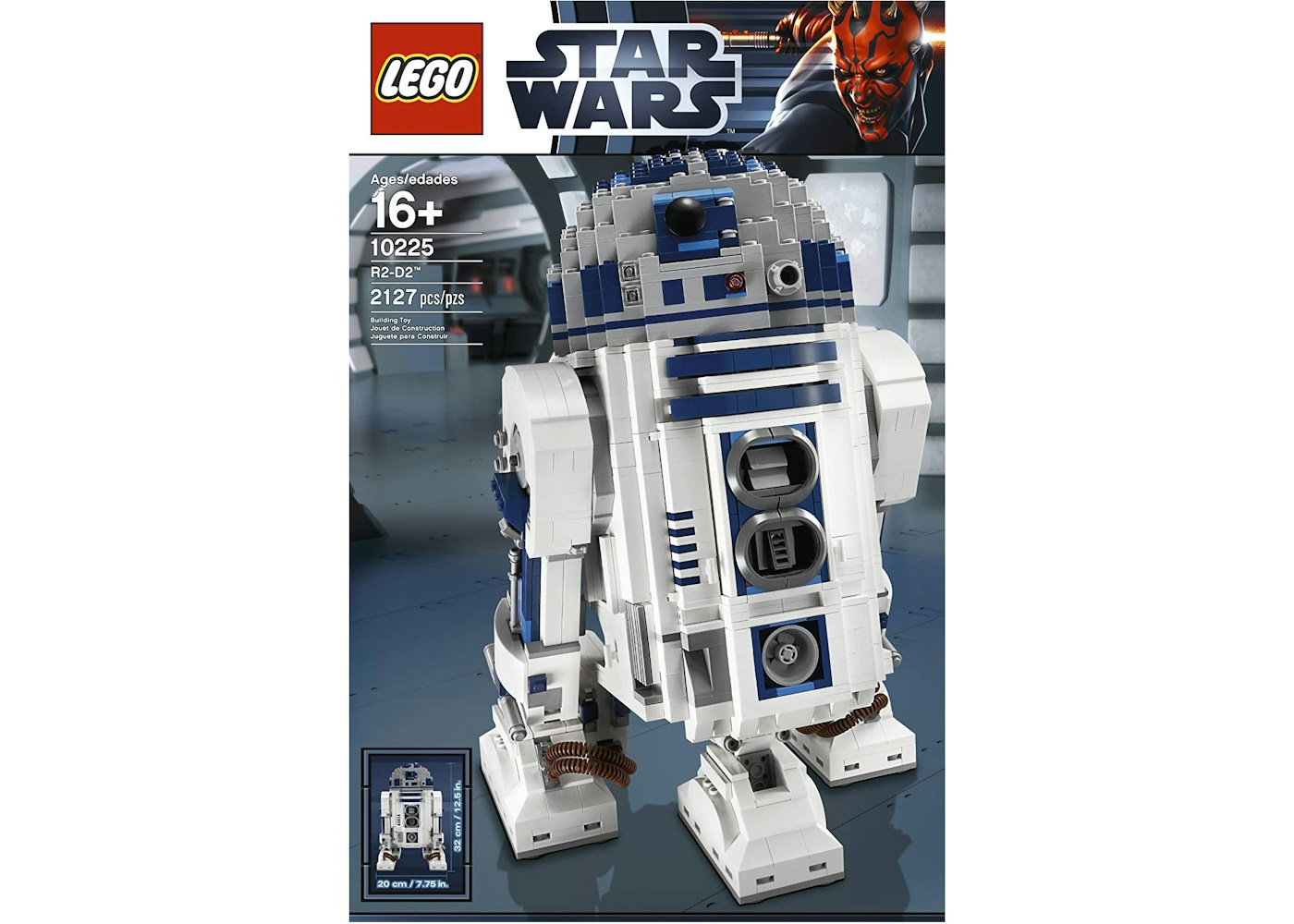



Lego Star Wars R2 D2 Set




Amazon Com Star Wars Droid Salt And Pepper Shakers Ceramic R2 D2 And R2q5 Add A Little Star Wars To Every Meal Kitchen Dining
< E H A Y> @ X S s @ @10 4 @ @0108 @ { o Y @ g i g @ @9 06 @ @0402 @ A p b ` @ @8 23 @ @0700 @ ɓ @ @8 09 @ @0806 @ a @ @7 12 @ @1006 @ FS @ @3 29 @ @1011 @ b c @ @ @ @ @ @3 22 @ @1108 @ a @ @ @ @3 15 @ @0403 @ G @ g i g @ @2 22 @ � · Find dy/dx y = x x e (2x 5) mention each and every step Find dy/dx (x) 1/2 (y) 1/2 = (a) 1/2 Mention each and every step If y = tan1 a/x log (xa/xa) 1/2, prove that dy/dx = 2a 3 /(x 4 – a 4) Mention each and every step Kindly Sign up for a personalised experience Ask Study Doubts;In the above fX;Y and fY are pmf's;




Amazon Com Star Wars Droid Salt And Pepper Shakers Ceramic R2 D2 And R2q5 Add A Little Star Wars To Every Meal Kitchen Dining
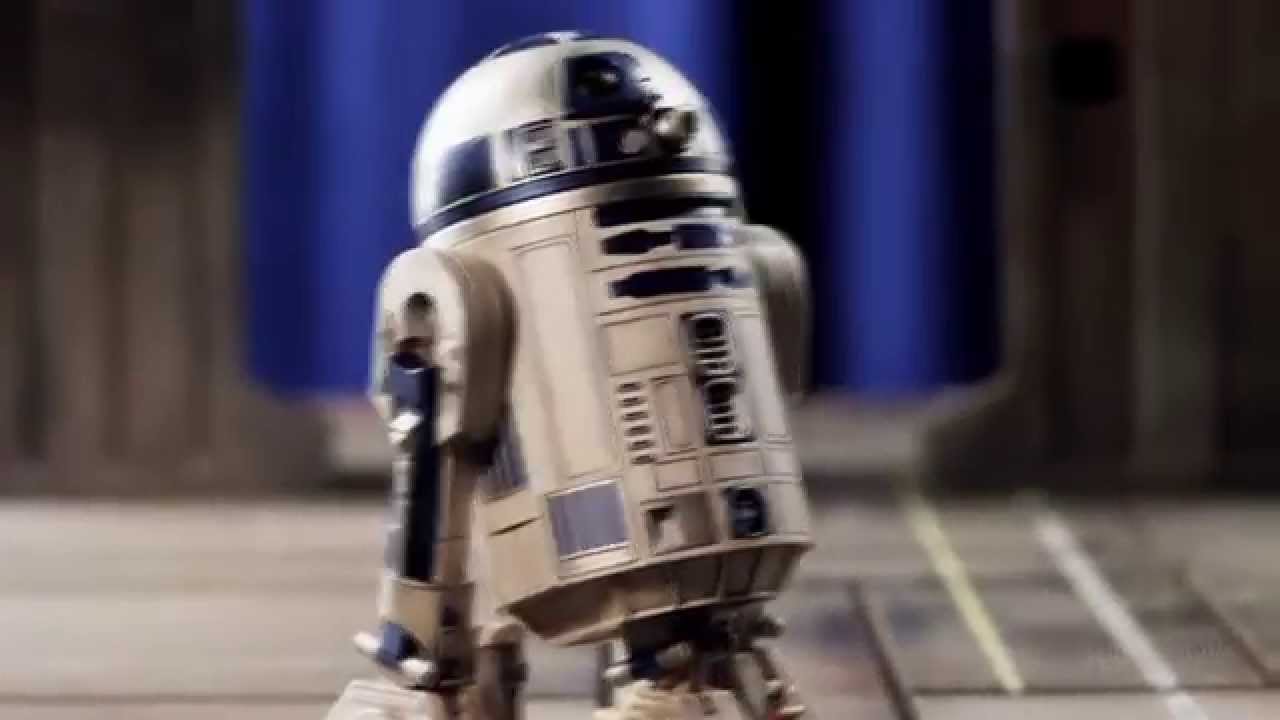



R2d2 Sixth Scale Figure Sideshow Collectibles Youtube
Then, once again, substitute the DGP, X Cu, for y, to get Var /O D E X0X/1X0X Cu/ X0X/1X0X Cu/ 0 D E CX0X/1X0u CX0X/1X0u 0 D E X0X/1X0u X0X/1X0u 0 D E hX0X/1X0uu0XX0X/1 i (5) (Note that the symmetric matrix X0X/ O1 is equal to its transpose) As with figuring the expected value of , we will take expectations conditional on X So we1,y = 0 and y = x The surface is defined by the function z = f(x,y) = √ 1−x2 Therefore, V = ZZ R zdxdy = Z 1 0 (Z x 0 √ 1−x2dy)dx = 1 3 6 (a) Let D(a) = {(x,y) x2 y2 ≤ a} Then ZZ D(a) e−(x2y2)dxdy = Z2π 0 Za e −r2rdrdθ = π(1−e a2) Therefore, lim a→∞ RR D(a) e−(x2y2)dxdy = π (b) Let D 1(a) = {(x,y) x,y ≥ 0, x2 y2 ≤ a} and D 2(a) = {(x,y) 0 ≤ x,y ≤ a} Note that Z Z D 1(a)Var(X Y) = E h (X Y)2 i −(EX Y)2 = E X2 2XY Y 2 −(EXEY) = E X 2 2EXYE Y 2 − h (EX) 2EXEY(EY) i = E X 2 −(EX) E Y2 −(EY) 2EXY−2EXEY = Var(X)Var(Y)2EXY−2EXEY But we've just seen that EXY = EXEY if X and Y are independent, so then Var(X Y) = Var(X)Var(Y) 3
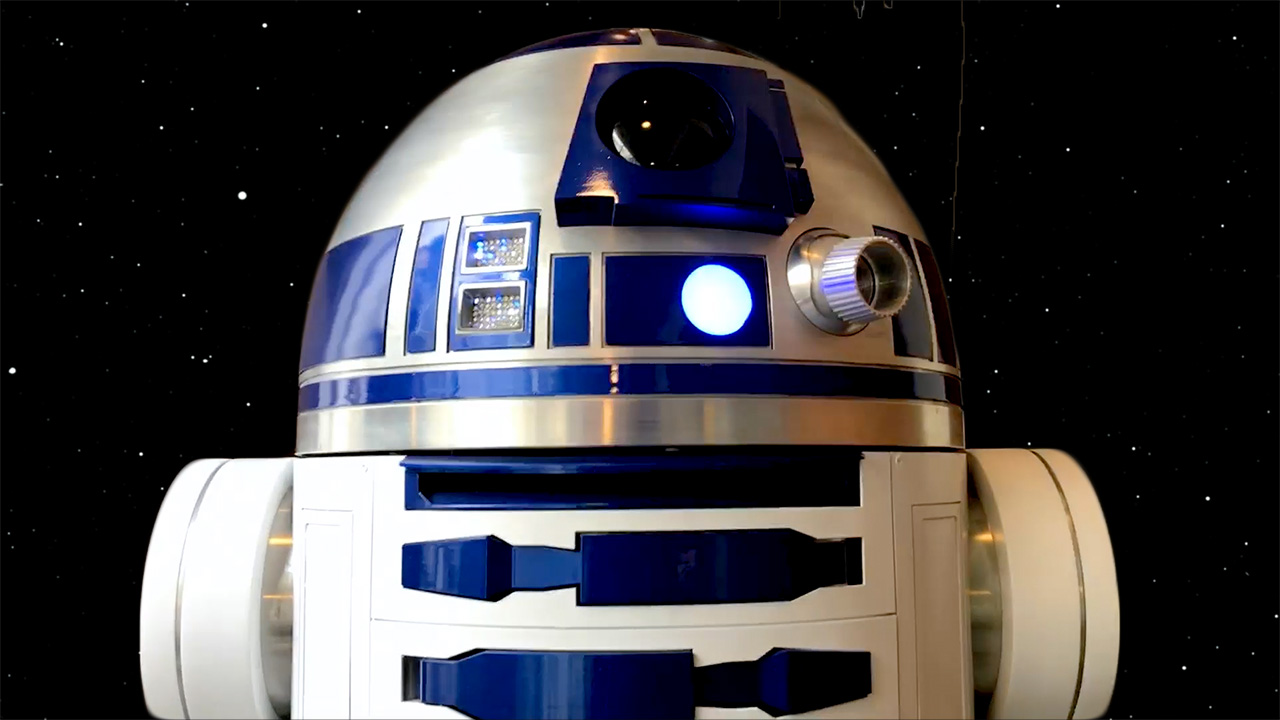



R2 D2 Takes You On A Tour Of Lucasfilm S Amazing Collection D23




R2 D2 C 3po 8 And Chopper Star Wars Pictures Star Wars 8 Star Wars Droids
1 दवे इं 2 दे वा इं 3From below, in part (c), we know that min(X,Y) is a geometric random variable mean pq −pq Therefore, Emin(X,Y) = 1 pq−pq, and we get Emax(X,Y) = 1 p 1 q − 1 pq −pq (c) What is Pmin(X,Y) = k?X ^ E H Y R2D2 ^ C v v l ^ E z X ^ R2D2 ~ j ( ܂ t) \2,980( ō ) 5,000 ȏ㊮ I e r ԑg ł Љ b 葛 R I 2 T t ( 艖 Z b g u ) ł B 傫 ڂ̃T C Y ̕ ̕ ɂ́A ̕ ܂ B
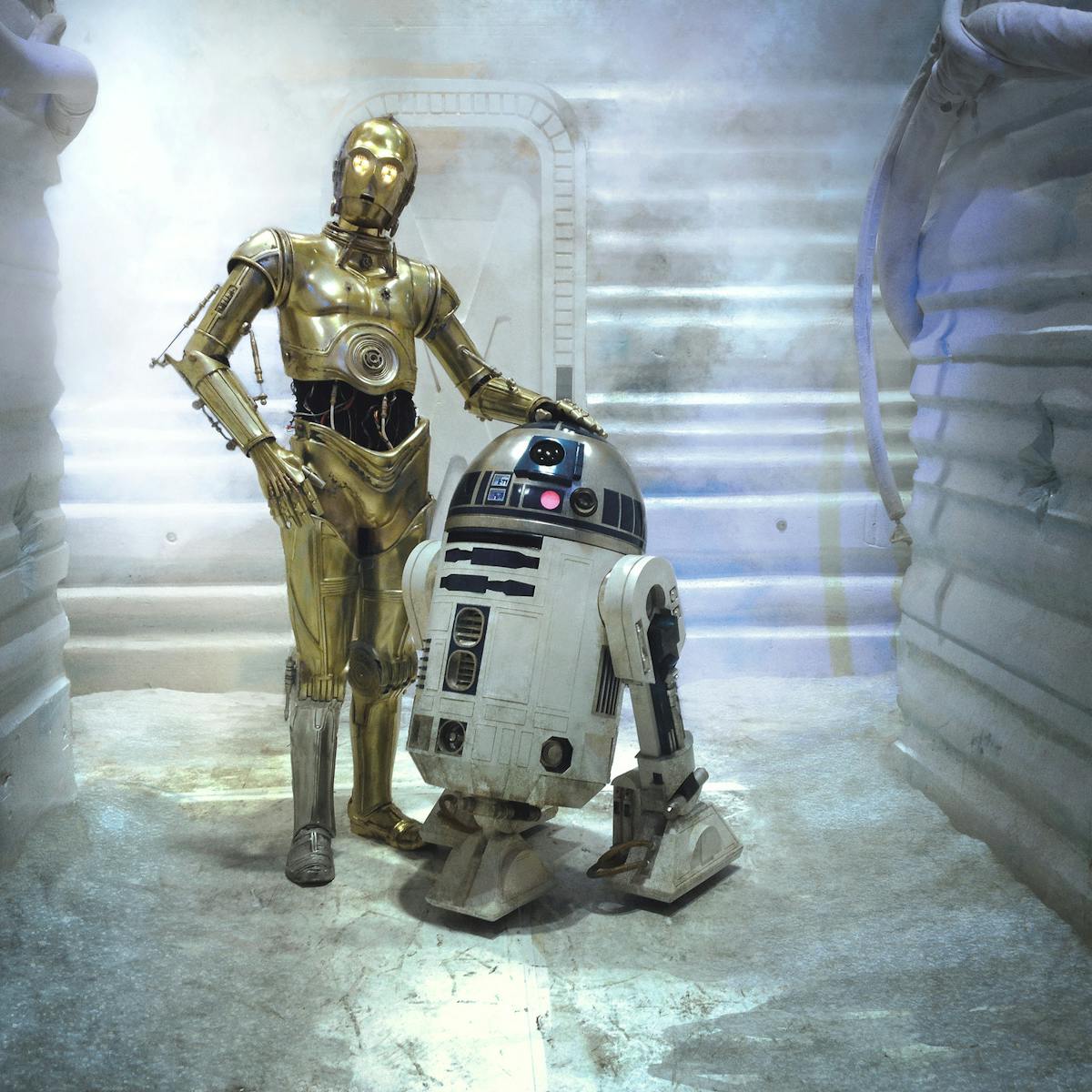



How Long Until We Can Build R2 D2 And C 3po



Tales To Astonish Star Wars
X ^ E H Y R2D2 g L O t b W K W F b g(R2D2) O b Y ① STAR WARS/Talking Fridge Gadget ystar X ^ E H Y R2D2 g L O t b W K W F b g(R2D2) O b Y ① STAR WARS/Talking Fridge Gadget ystarwars_y z @ i F @0 ~ @ r F @10 @ ^ @ ϕ F @440 _ @ ̔ X F @iPhone P X J o O b Y iPlus @ ڍׂ́A @ 0247 X V X ^ E H Y O b Y / 3D } O J b v R2D2 @ i F @0 ~ @ r F @2X2 Dy Y (X Y) Dx = 0 CBSE CBSE (Science) Class 12 Question Papers 1851 Textbook Solutions Important Solutions 4563 Question Bank Solutions Concept Notes & Videos 735 Time Tables 18 Syllabus Advertisement Remove all ads X2 Dy Y (X Y) Dx = 0 Mathematics Advertisement Remove all ads Advertisement Remove all ads AdvertisementLet y = y (x) be the solution of the differential equation sin x d x d y y cos x = 4 x, x ∈ (0, π) If y (2 π ) = 0, then y (6 π ) is equal to Hard View solution




Star Wars R2 D2 Premium Format
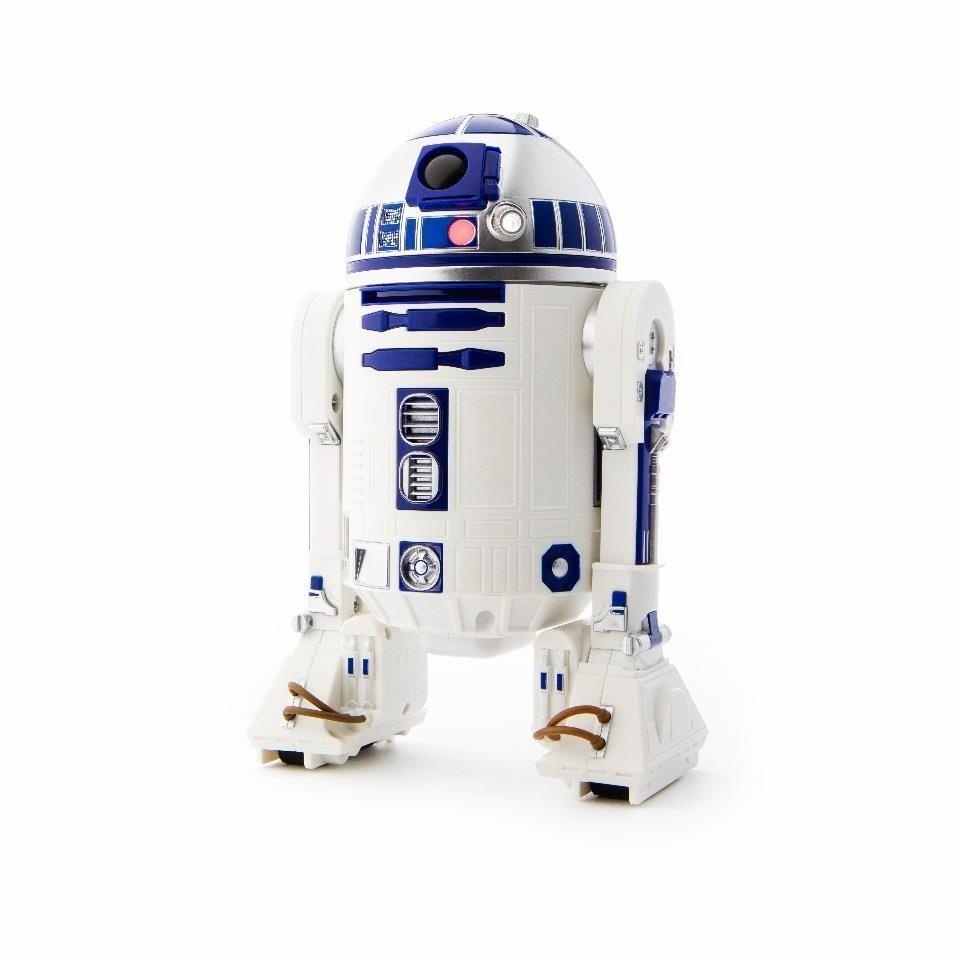



Buy Sphero Star Wars R2 D2 App Enabled Droid R1row At Affordable Prices Price 243 Usd Free Shipping Real Reviews With Photos Joom
The interval a;b with d(x;y) = jx yjis a subspace of R 2 The unit circle f(x 1;x 2) 2R2 x2 1 x 2 2 = 1gwith d(x;y) = p (x 1 y 1) (x 2 y 2)2 is a subspace of R2 3 The space of polynomials Pis a metric space with any of the metrics inherited from Ca;b above 13 De nition 3 Let (X;d) be a metric space, let x2Xand let r>0 The open ball centred at x, with radius r, is the set B(x;r) = fy2X d(x;y)Sign Up Verify your number toClick here👆to get an answer to your question ️ Find HCF of 81 and 237 Also express it as a linear combination of 81 and 237 ie, HCF of 81,237 = 81x 237 y for some x, y Note Values of x and y are not unique
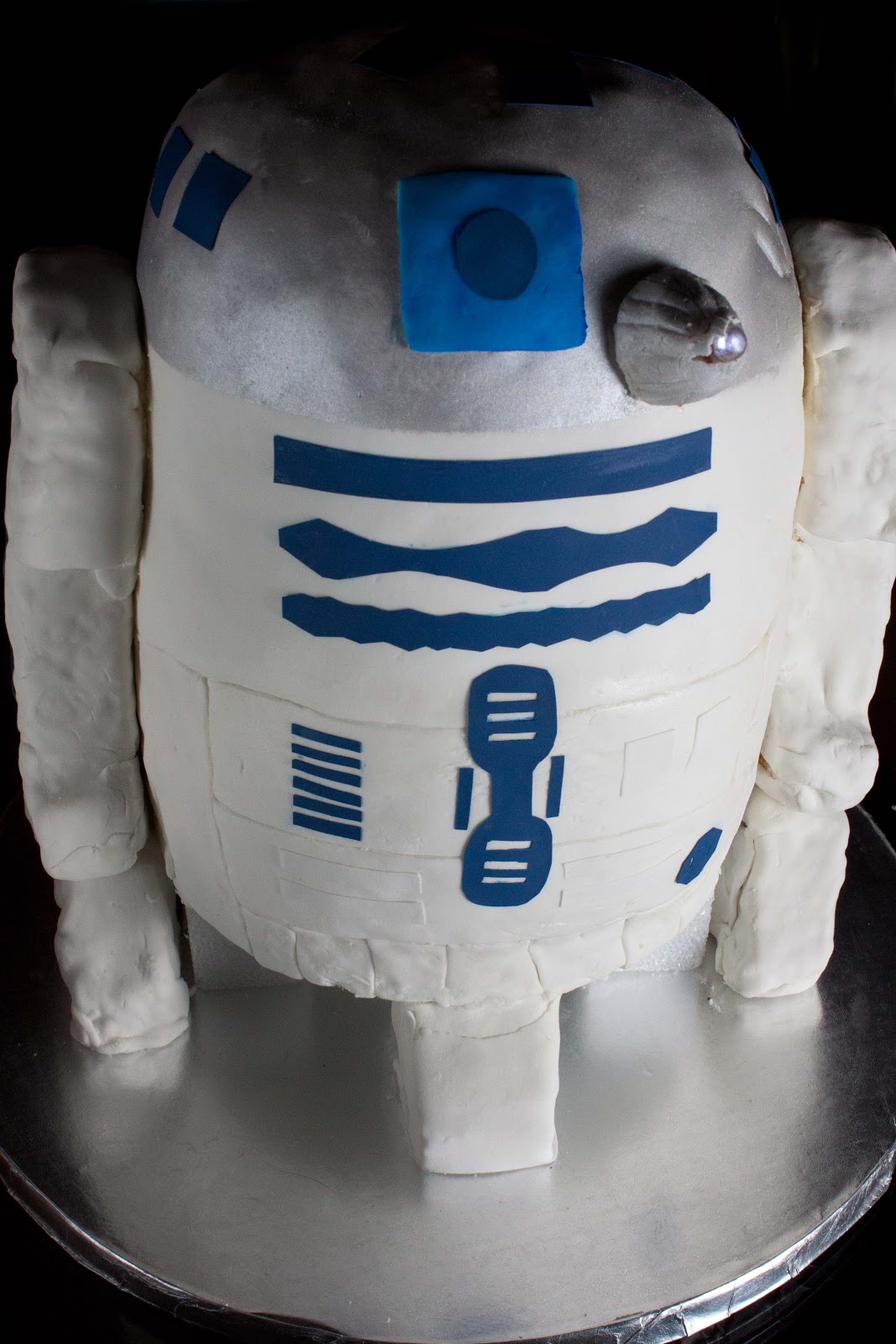



Fat And Happy Blog Star Wars R2d2 Birthday Cake
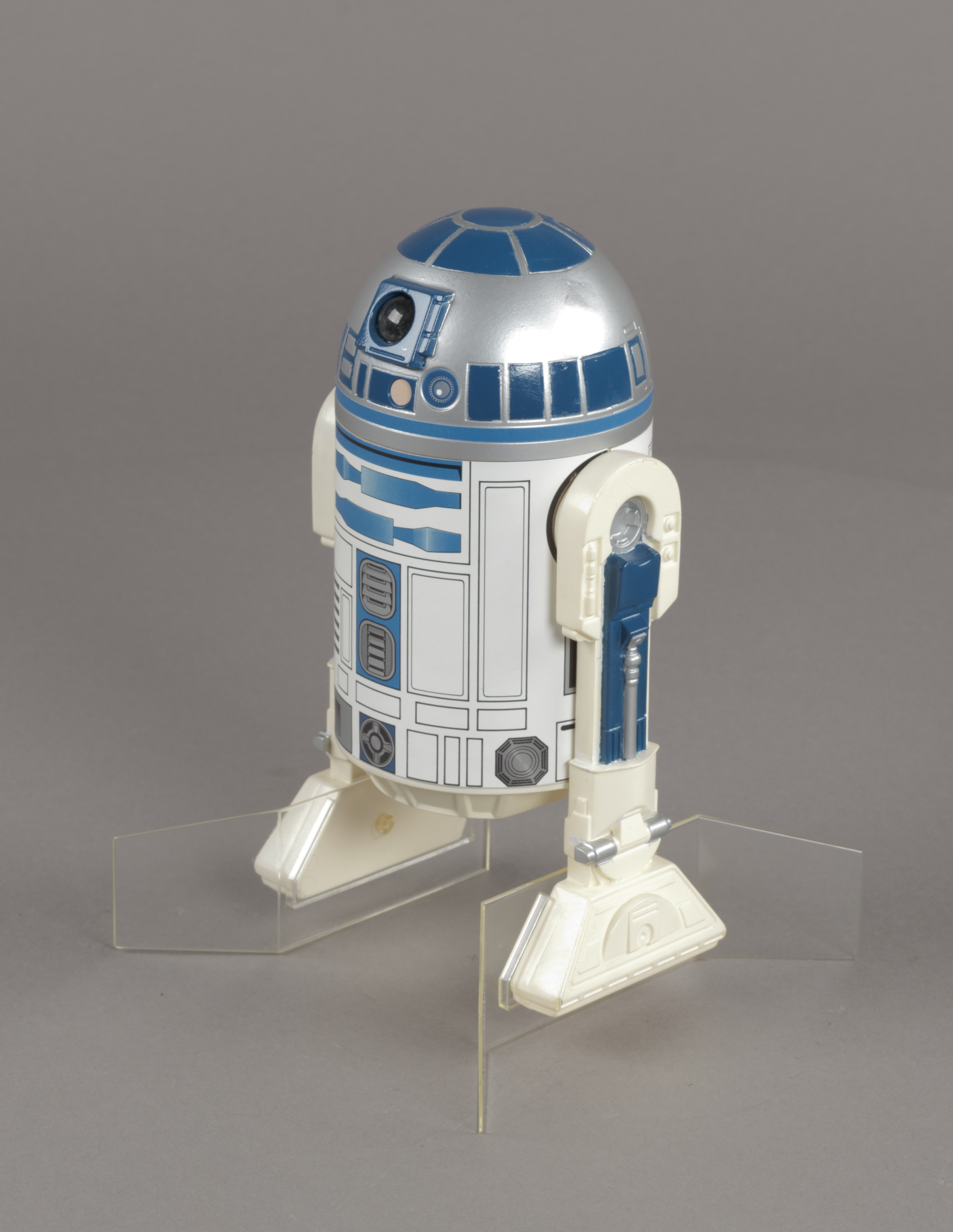



How Star Wars Revolutionized Entertainment National Air And Space Museum
June 24, 16 531am #2 real75 the roots are 1 and 1 Your answer has the rootIn probability theory, the expected value of a random variable, denoted or , is a generalization of the weighted average, and is intuitively the arithmetic mean of a large number of independent realizations of The expected value is also known as the expectation, mathematical expectation, mean, average, or first momentExpected value is a key concept in economics, finance, and manyBias(x)=L(y*,y m) • Define "variance of learner" Var(x)=E DL(y m,y) • Define "noise for x" N(x) = E tL(t,y*) Claim E D,tL(t,y) = c 1N(x)Bias(x)c 2Var(x) where c 1=Pr D y=y* 1 c 2=1 if y m=y*, 1 else m=D Domingos, A Unified BiasVariance Decomposition and its Applications, ICML 00 For 0/1 loss, the main prediction is
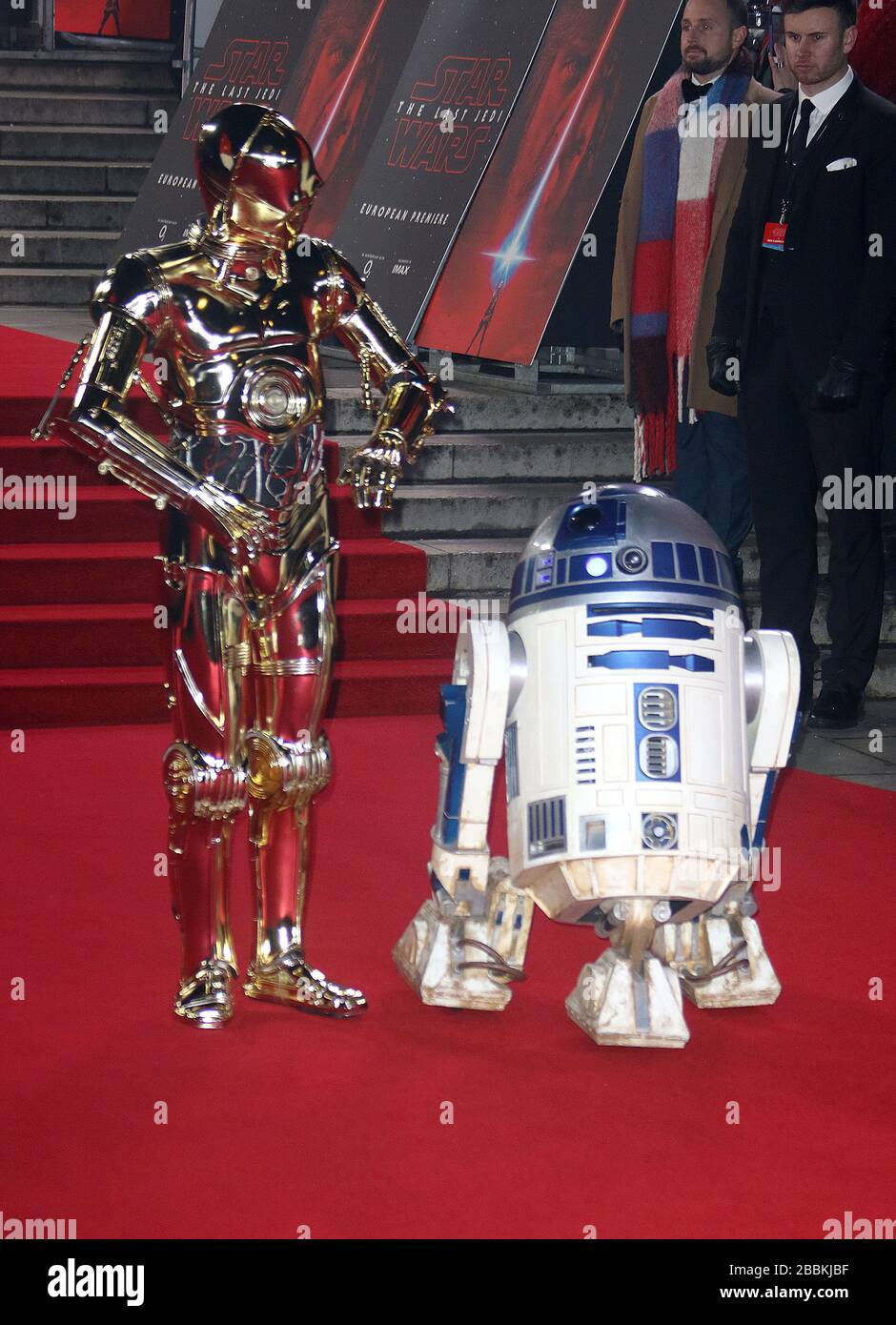



C3 Po High Resolution Stock Photography And Images Alamy




Hallmark Christmas Ornament Star Wars R2 D2 Ornament Buy Online In Guyana At Guyana Desertcart Com Productid
Methods of Solving First Order, First Degree Differential Equations Homogeneous Differential Equations video tutorial ;WATCH STATION INTERNATIONAL b E H b ` X e V C ^ i V i i Y j ̎ v w 邱 Ƃ ł ܂ B z i ꕔ n j p ܂ B WATCH STATION INTERNATIONAL ̎ v i Y j c P ł ܂ B x 10 25 OK ڍ
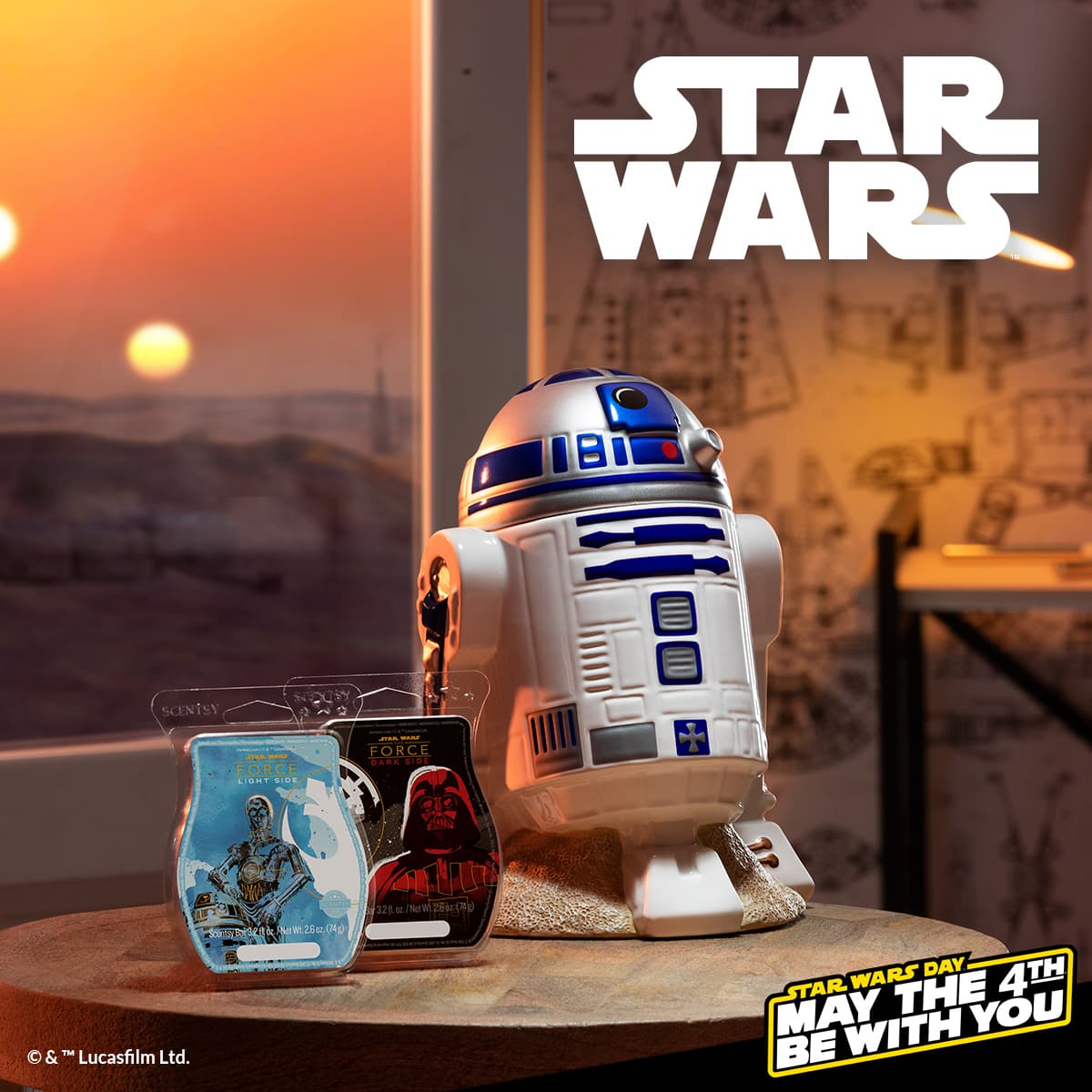



Star Wars Scentsy Collection R2 D2 Scentsy Warmer Shop Now Incandescent Scentsy Us
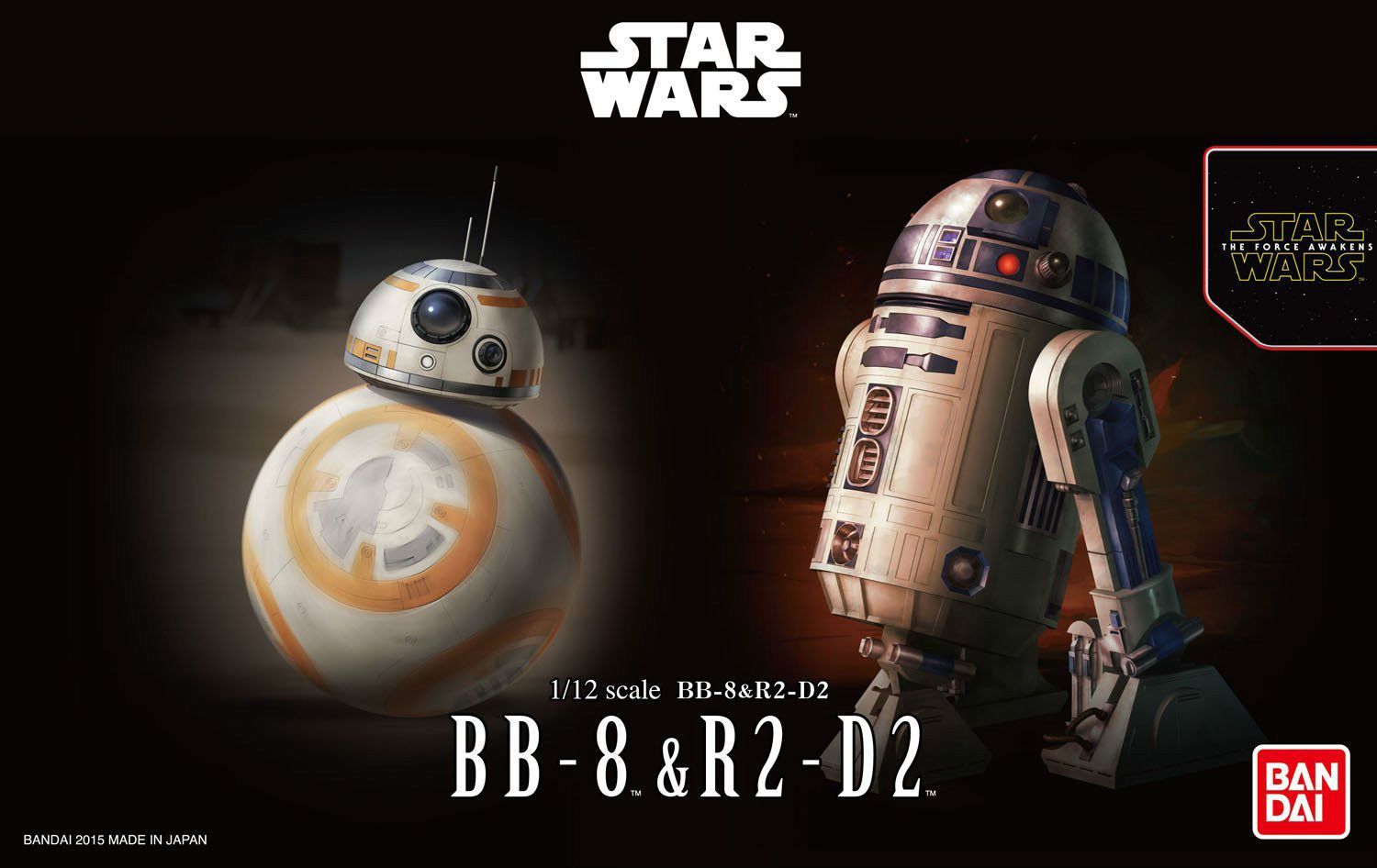



Tactics Plastics Bandai Star Wars Model Kit 8 R2 D2 Assemble And Review
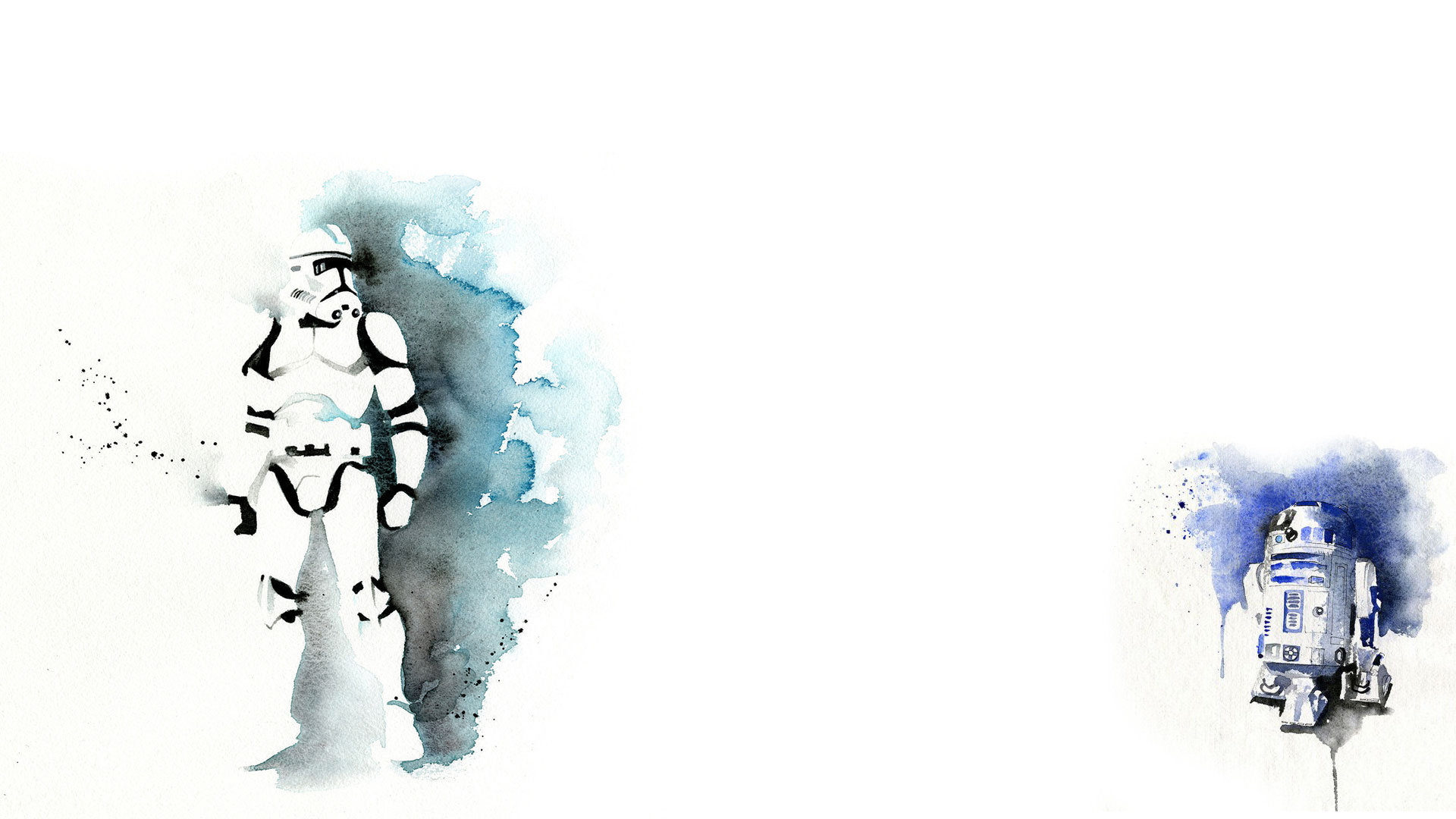



Free R2 D2 High Quality Wallpaper Id For Full Hd 19x1080 Desktop
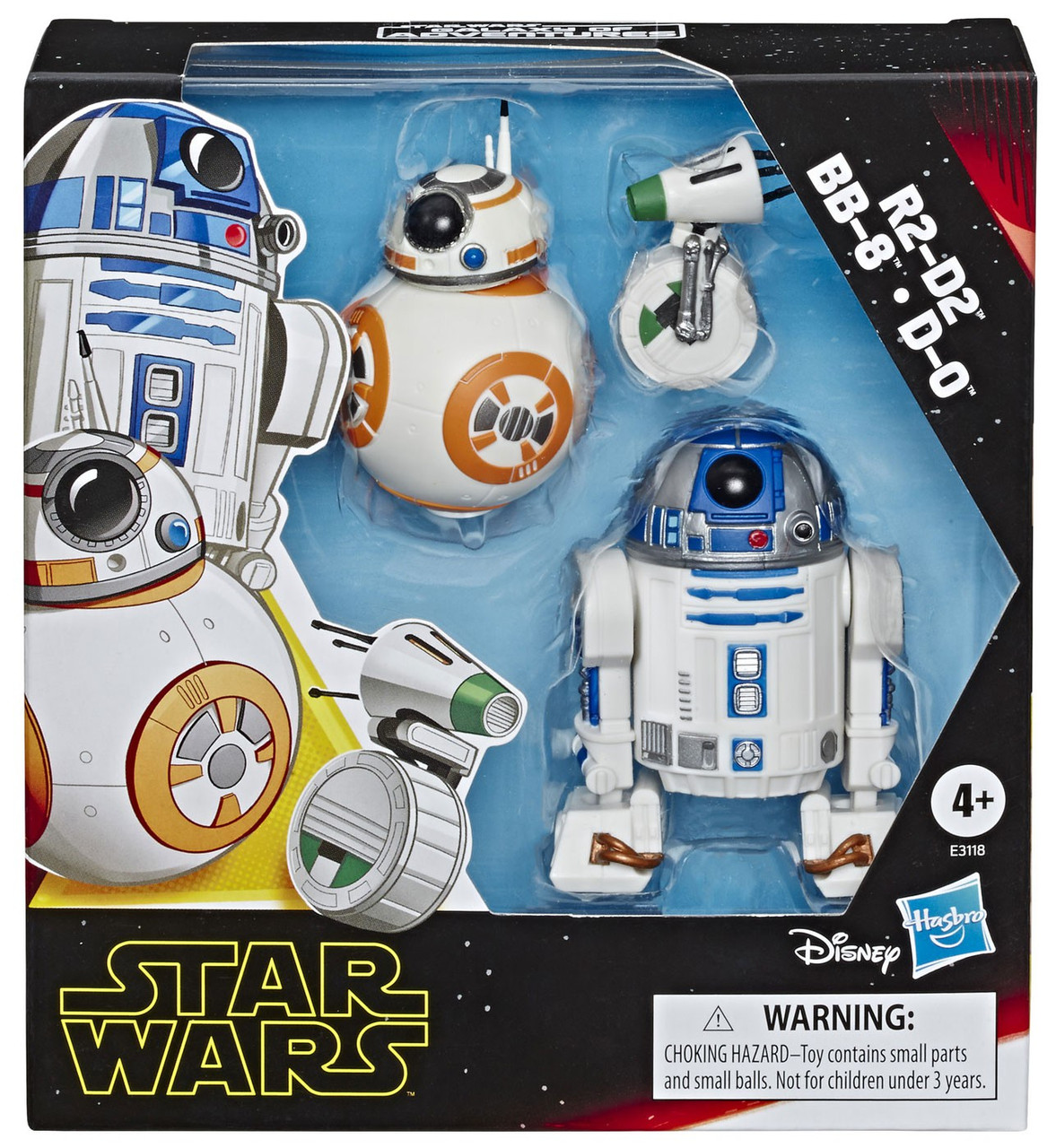



Star Wars The Rise Of Skywalker Galaxy Of Adventures R2 D2 8 D O 5 Action Figure 3 Pack Hasbro Toys Toywiz
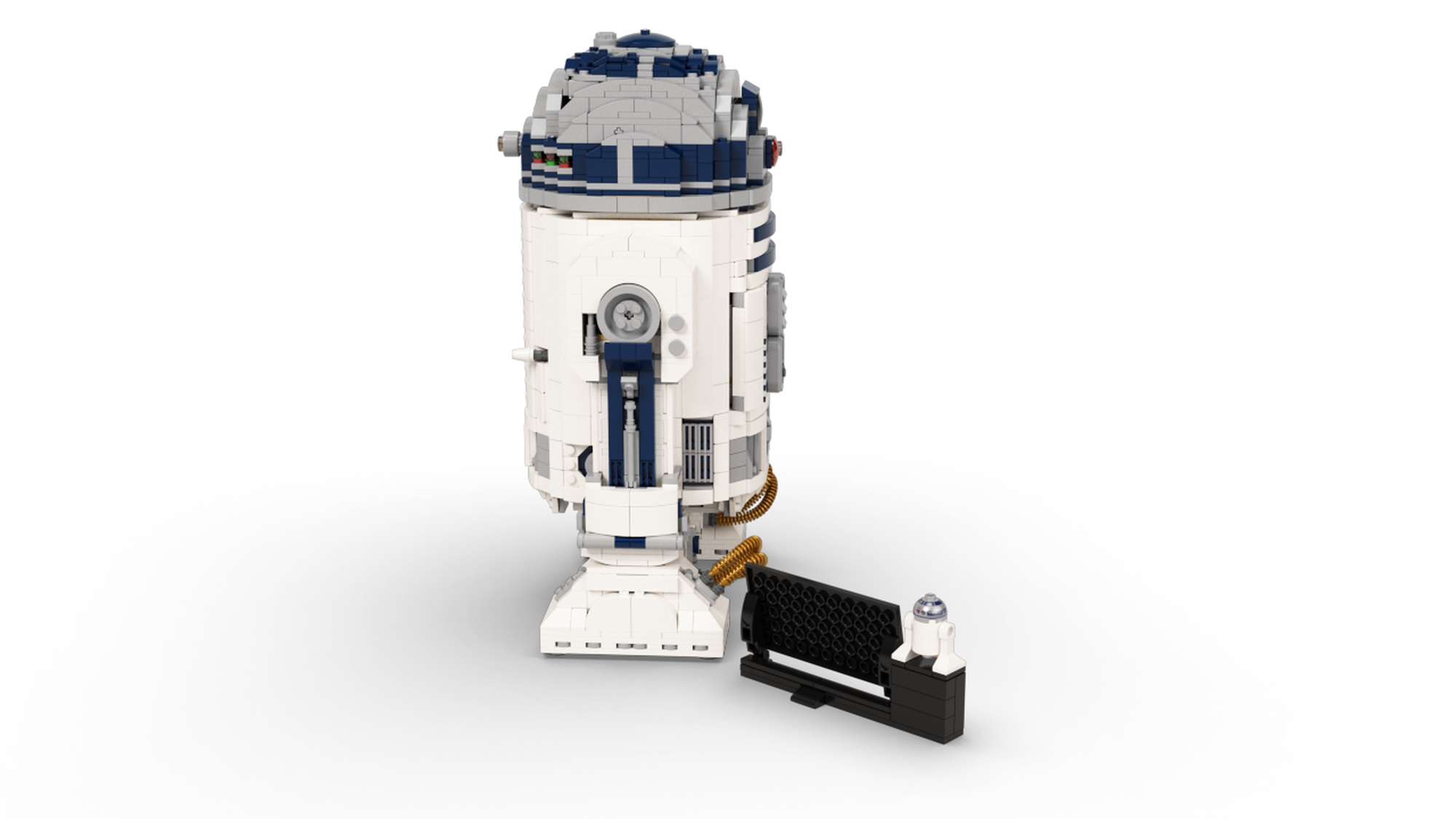



Lego R2 D2 Brickshop Lego En Duplo Specialist




Cufflinks Inc 3d Star Wars R2 D2 Cuff Links Neiman Marcus




Star Wars The Force Awakens R2 D2 Hot Toys Machinegun




Best Star Wars Gifts 21
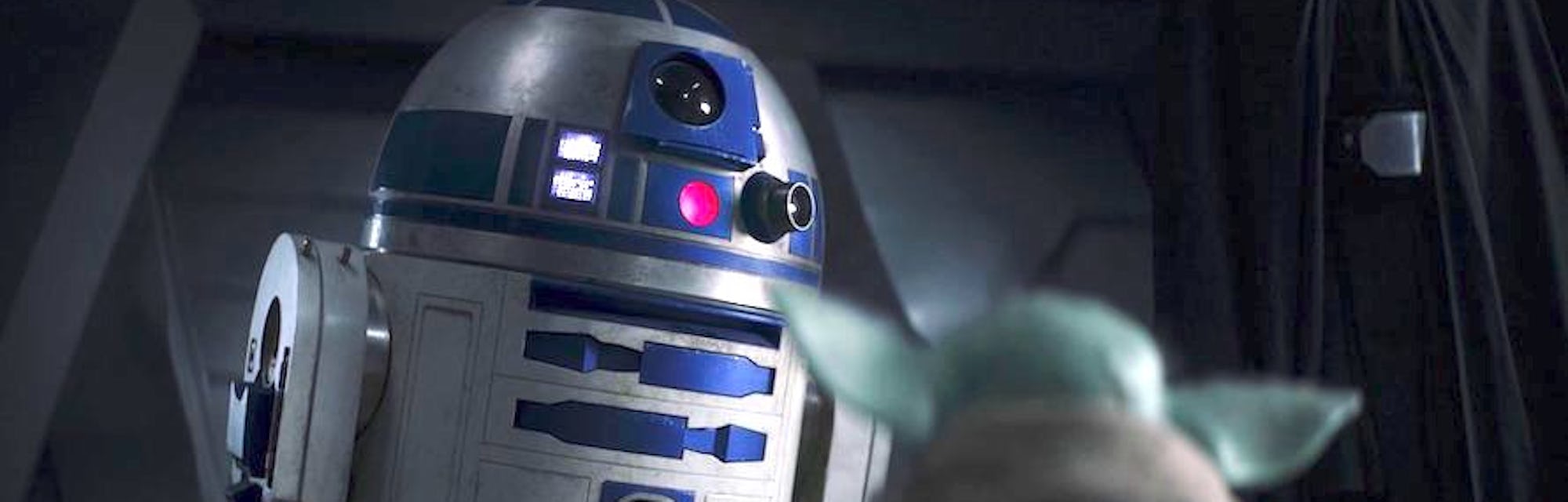



Mandalorian R2 D2 May Confirm A Mind Blowing Star Wars Fan Theory
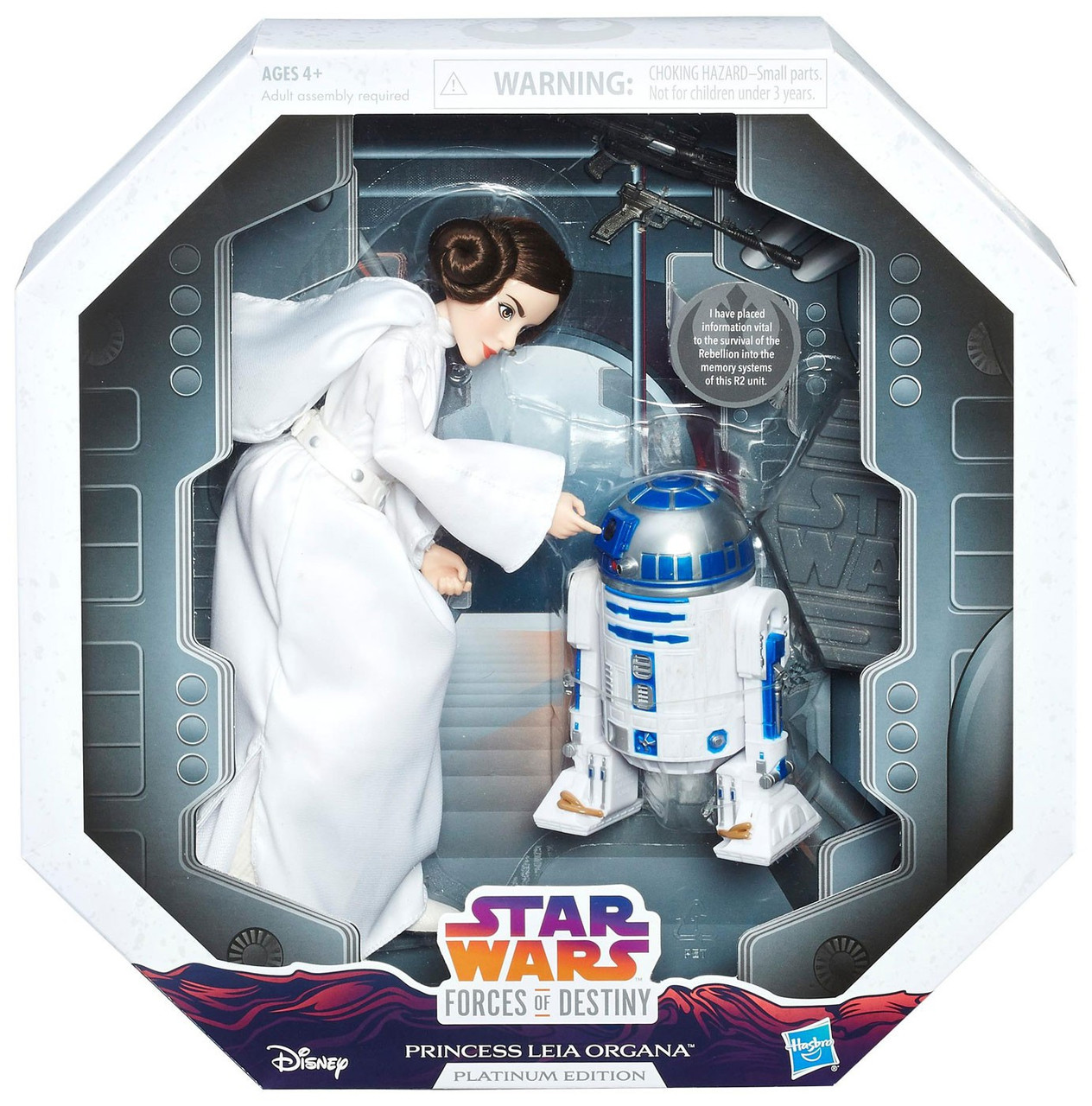



Star Wars Forces Of Destiny Princess Leia Organa R2 D2 Figure 2 Pack Platinum Edition Hasbro Toys Toywiz



R2 D2 Wookieepedia Fandom
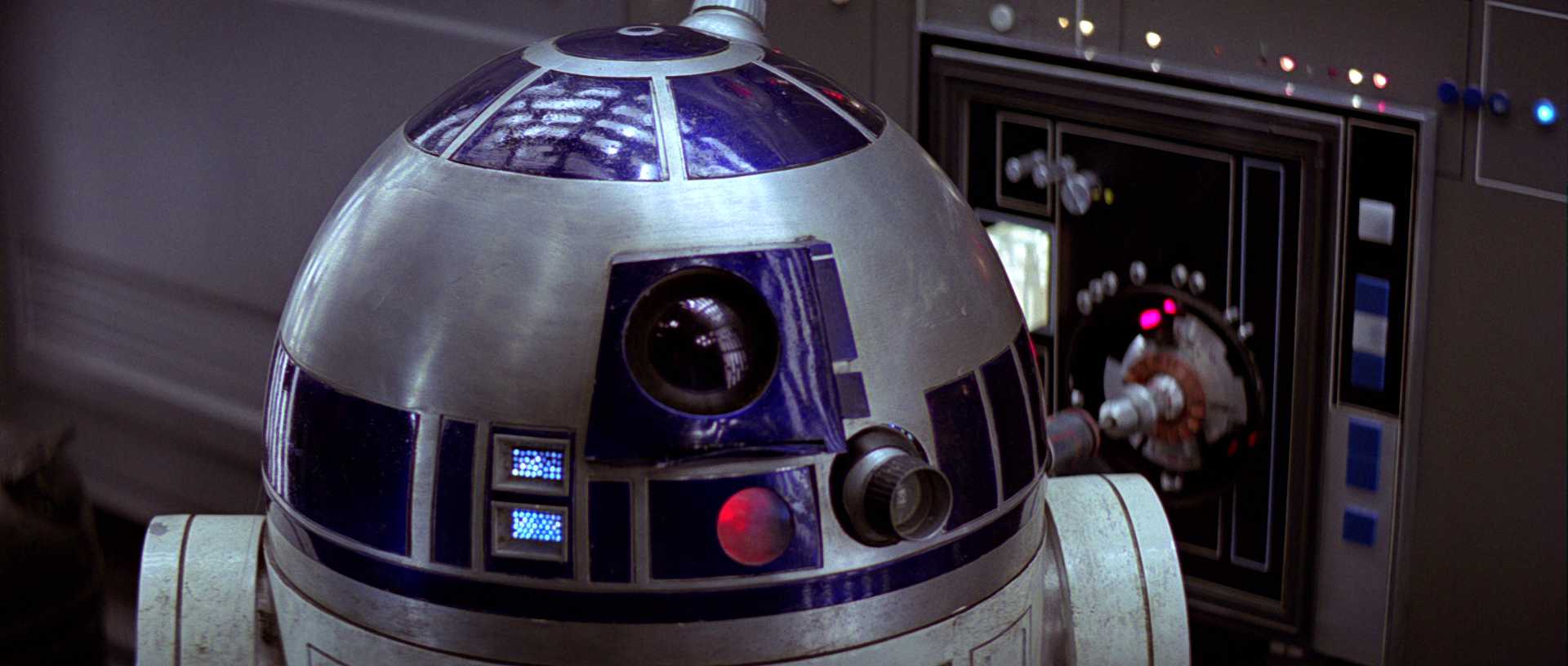



R2 D2 Wookieepedia Fandom
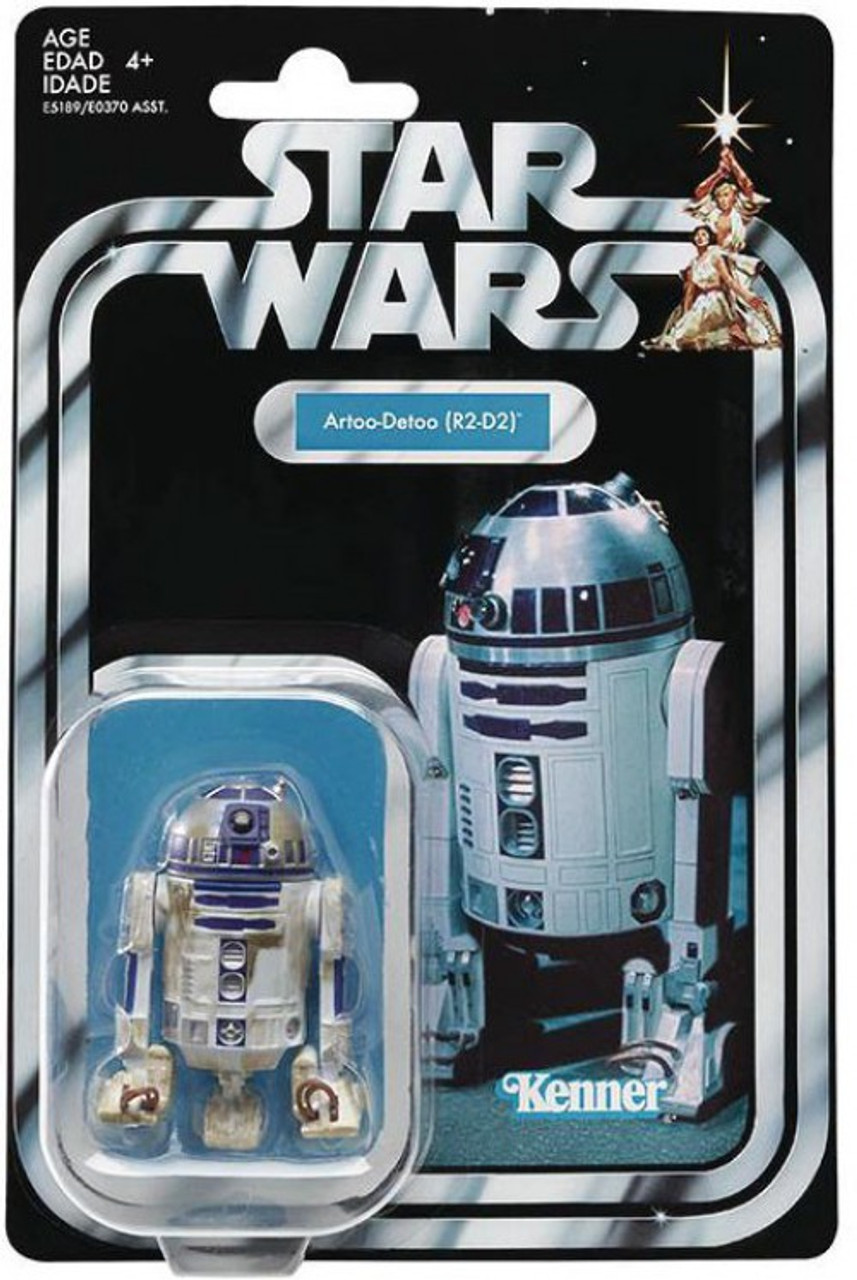



Star Wars A New Hope Vintage Collection Wave 21 R2 D2 3 75 Action Figure Hasbro Toys Toywiz




Sphero Launches New Programmable Star Wars Robots Including R2 D2




R2 D2 Skilled Starship Mechanic Star Wars Buddies Episodes I Thru Viii Plus Others The Kenner Vintage Collection Rare Vintage 1997 Now And Then Collectibles




The Blot Says Nycc 16 Exclusive Star Wars C 3po R2 D2 Laser Engraved Prints By Joshua Budich X Spoke Art
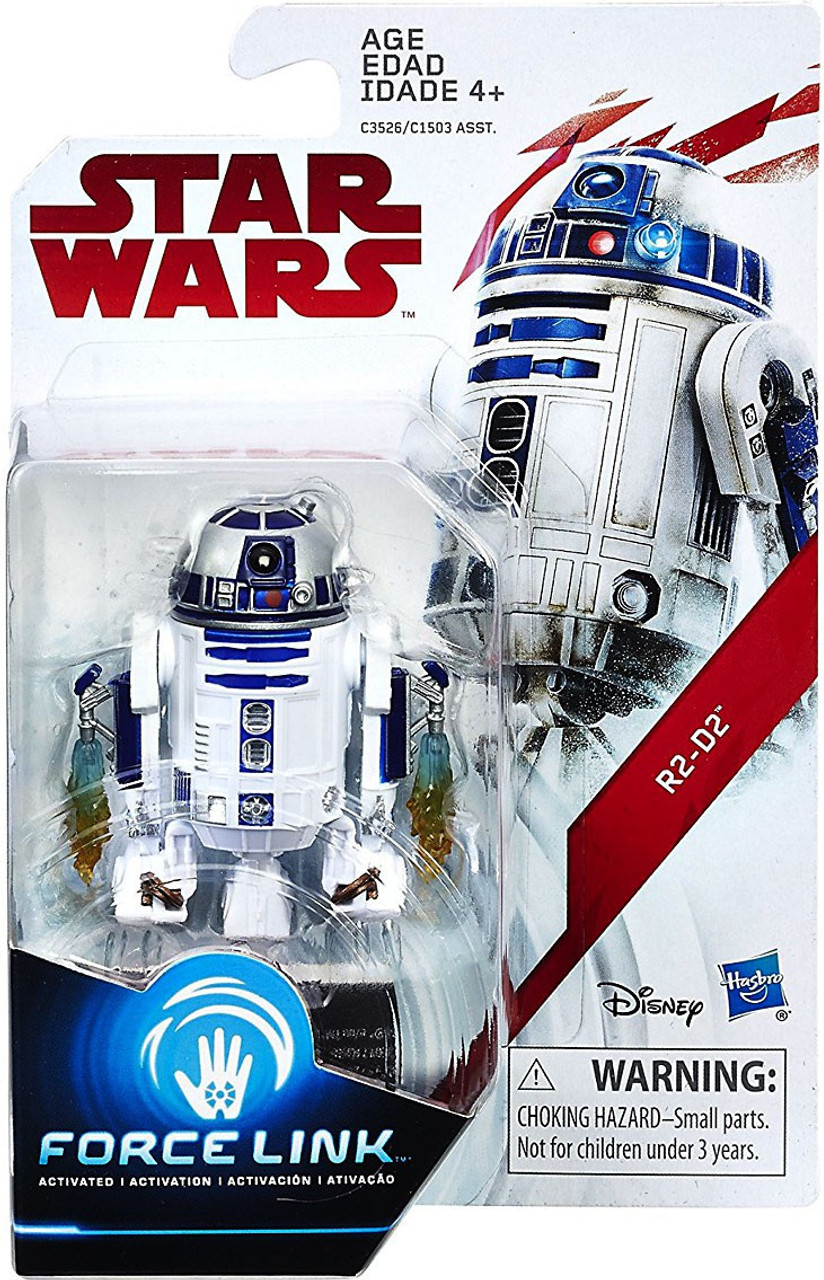



Star Wars The Last Jedi Force Link Orange Series Wave 2 R2 D2 3 75 Action Figure Hasbro Toys Toywiz




Toys Games Action Figures New S H Figuarts Star Wars R2 D2 A New Hope 90mm Abs Pvc Action Figure F S




U R2 Cute Diy R2 D2 Costume May The Fourth Be With You Party
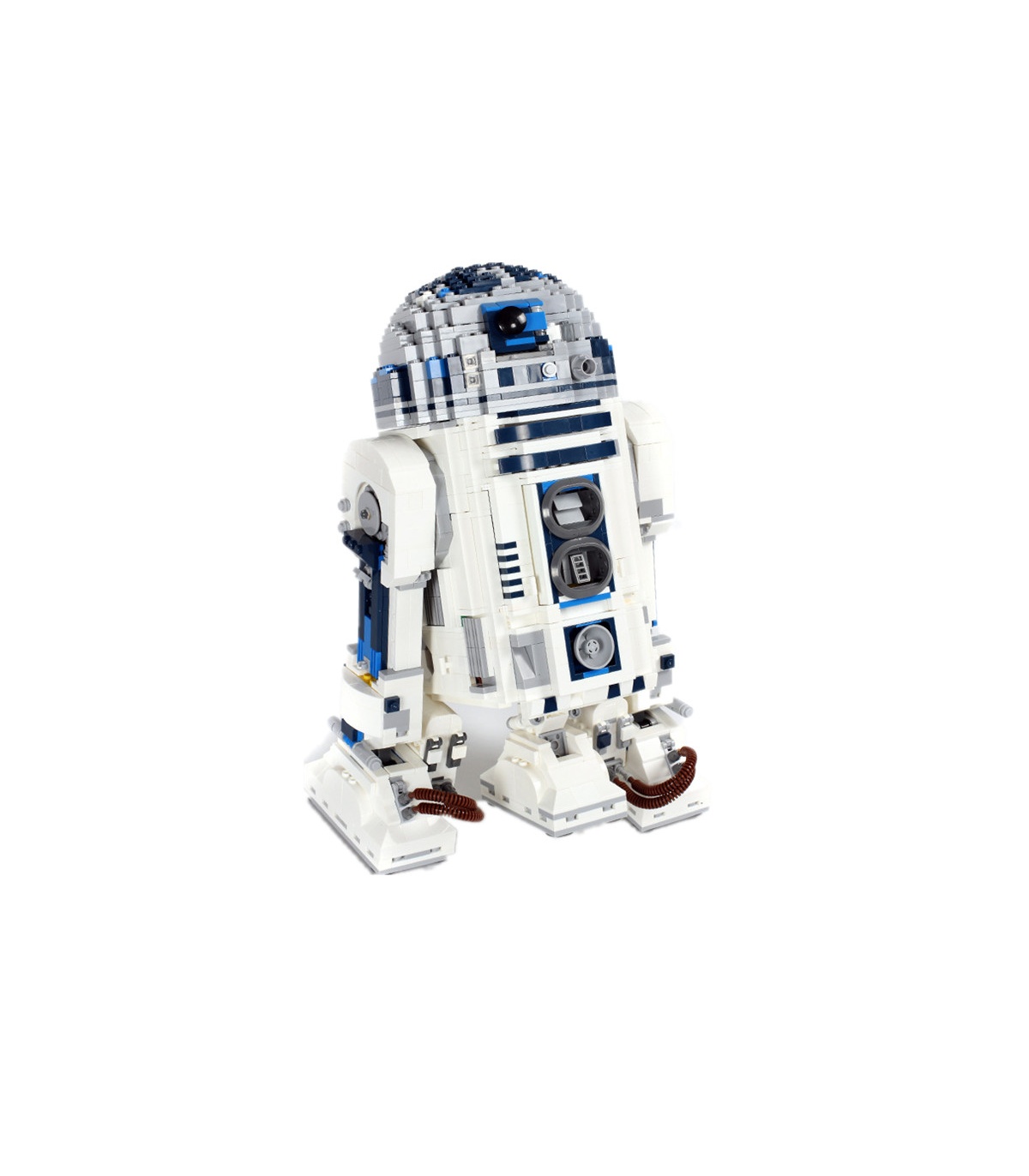



Custom Star Wars R2 D2 Compatible Building Bricks Toy Set 2127 Pieces Buildingtoystore Com
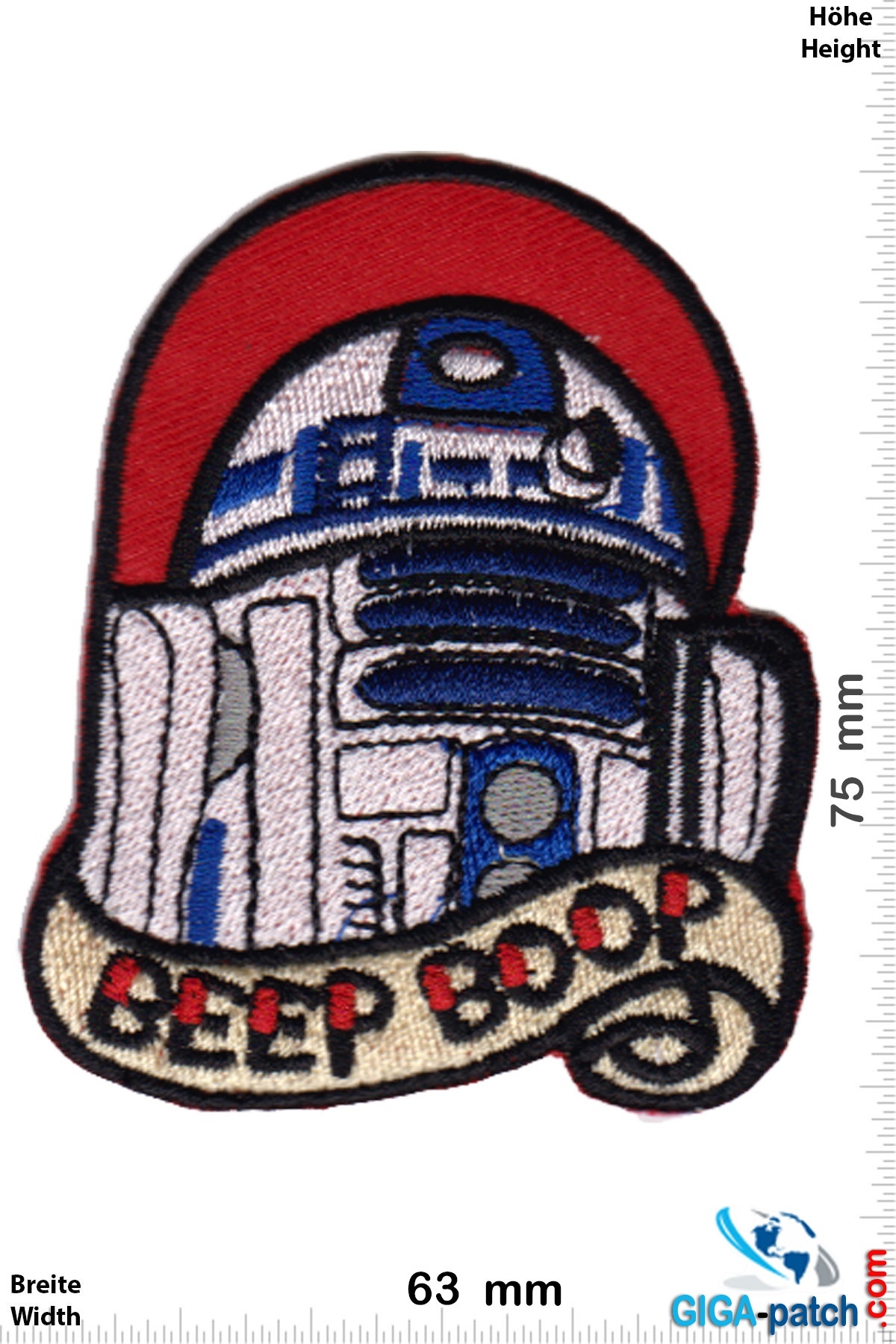



Star Wars Starwars R2 D2 Artoo Detoo Beep Boop Patch Back Patches Patch Keychains Stickers Giga Patch Com Biggest Patch Shop Worldwide




Amazon Com Bandai Hobby Star Wars 1 12 Plastic Model 8 R2 D2 Star Wars Toys Games




Omg It S R2d2 T Shirt 24h Delivery Getdigital




Artstation R2d2 Render Justin Mccollum Star Wars Images Star Wars Star Wars Art
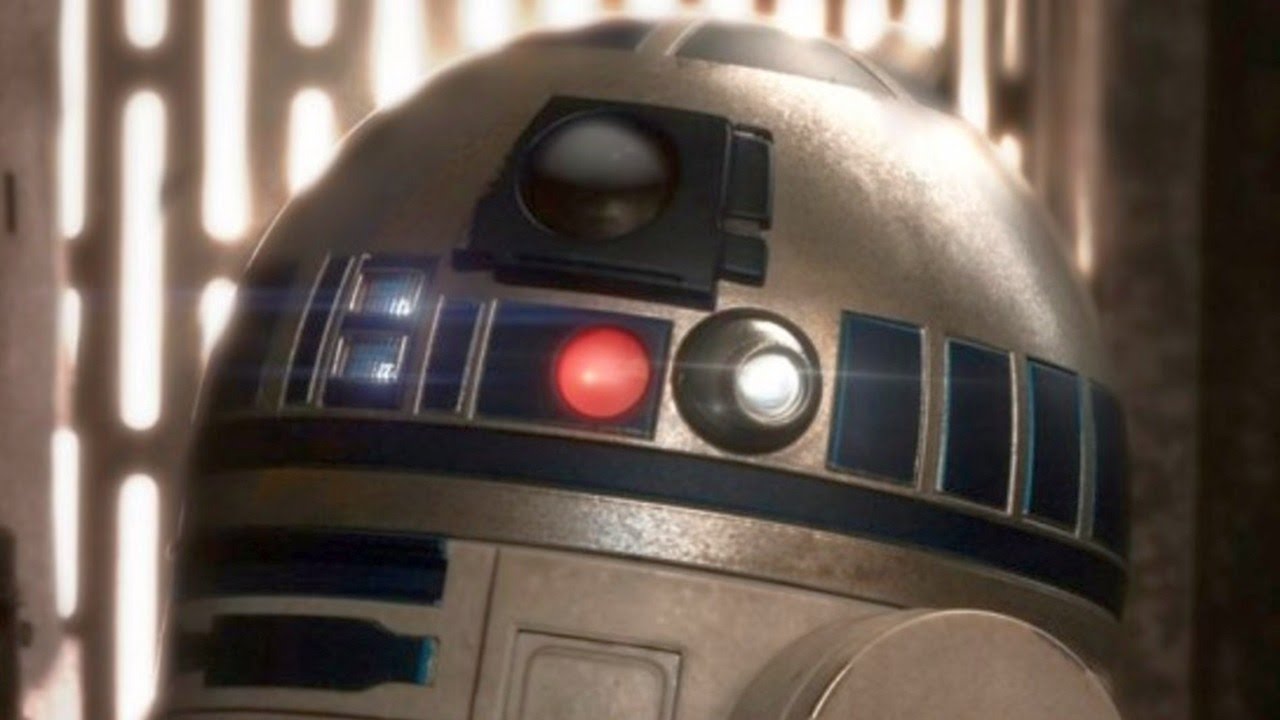



Every Time R2 D2 Saves The Day Youtube




Amazon Com Cardboard People R2d2 C3po Life Size Cardboard Cutout Standup Star Wars Classics Retouched Home Kitchen




Star Wars R2 D2 Rocket Booster Ver Bandai Star Wars 1 12 Plasticmodel Buy Online In Faroe Islands At Faroe Desertcart Com Productid
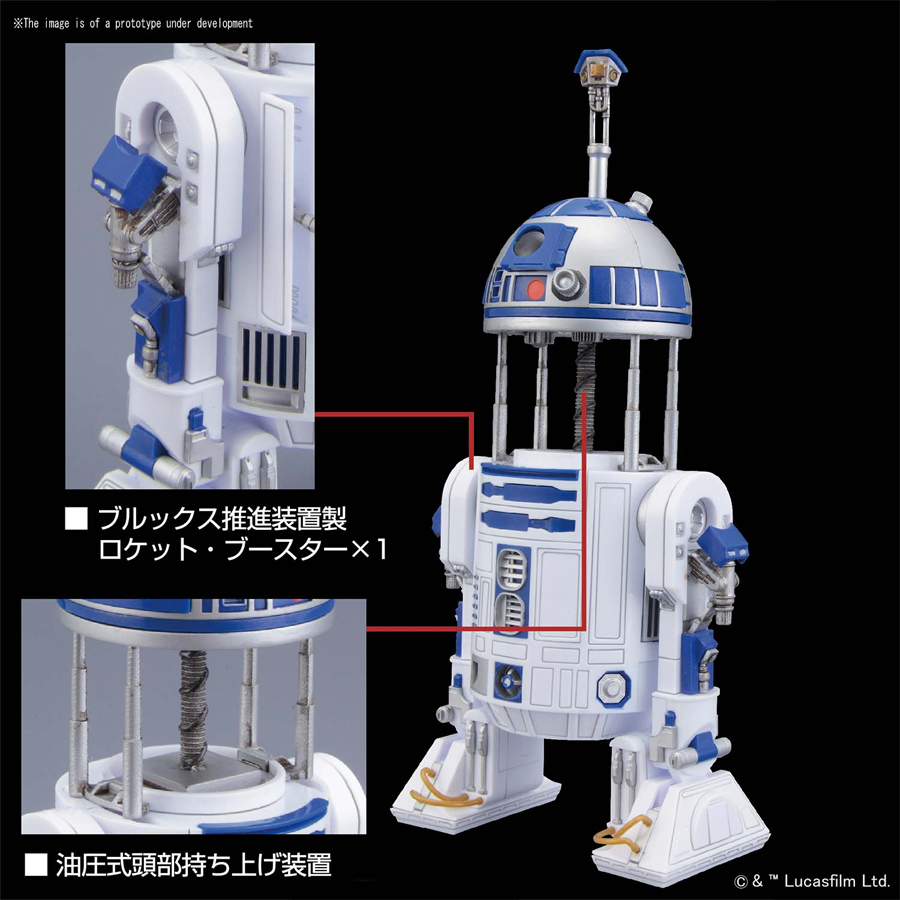



Star Wars Droid Collection R2 D2 Rocket Booster Version 1 12 Scale Model Kit Star Wars Droid Collection R2 D2 Rocket Booster Version 1 12 Scale Model Kit 26wba71 24 99 Monsters In Motion Movie




The Comprehensive Guide To Building A Realistic R2 D2 Replica Make




Star Wars The Force Awakens R2 D2 Hot Toys Machinegun




Amazon Com Star Wars Episode 1 R2 D2 Carryall Playset With Exclusive Destroyer Droid Figure Toys Games




The Comprehensive Guide To Building A Realistic R2 D2 Replica Make
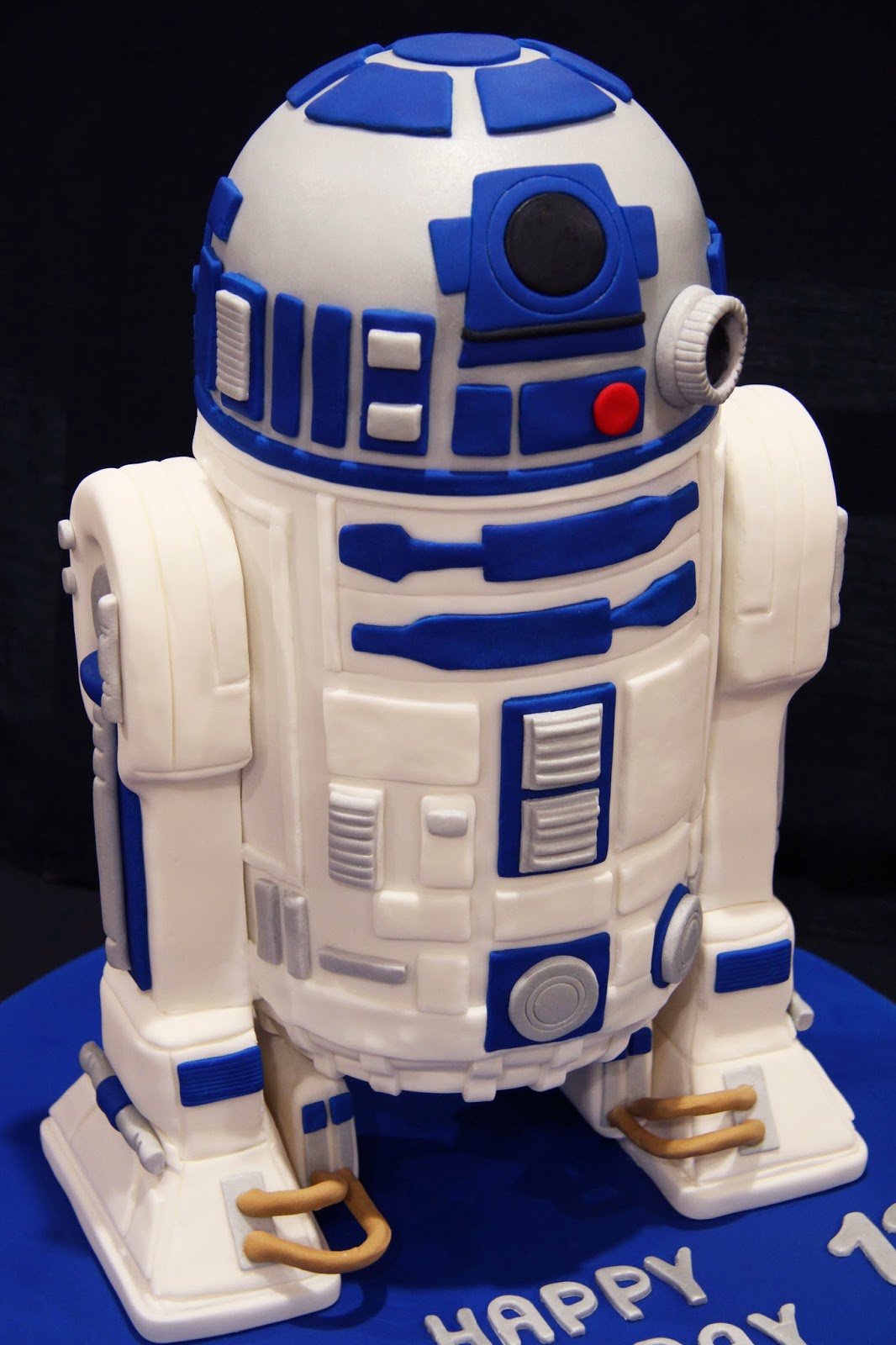



Leonie S Cakes And Parties Star Wars Party R2d2 Cake
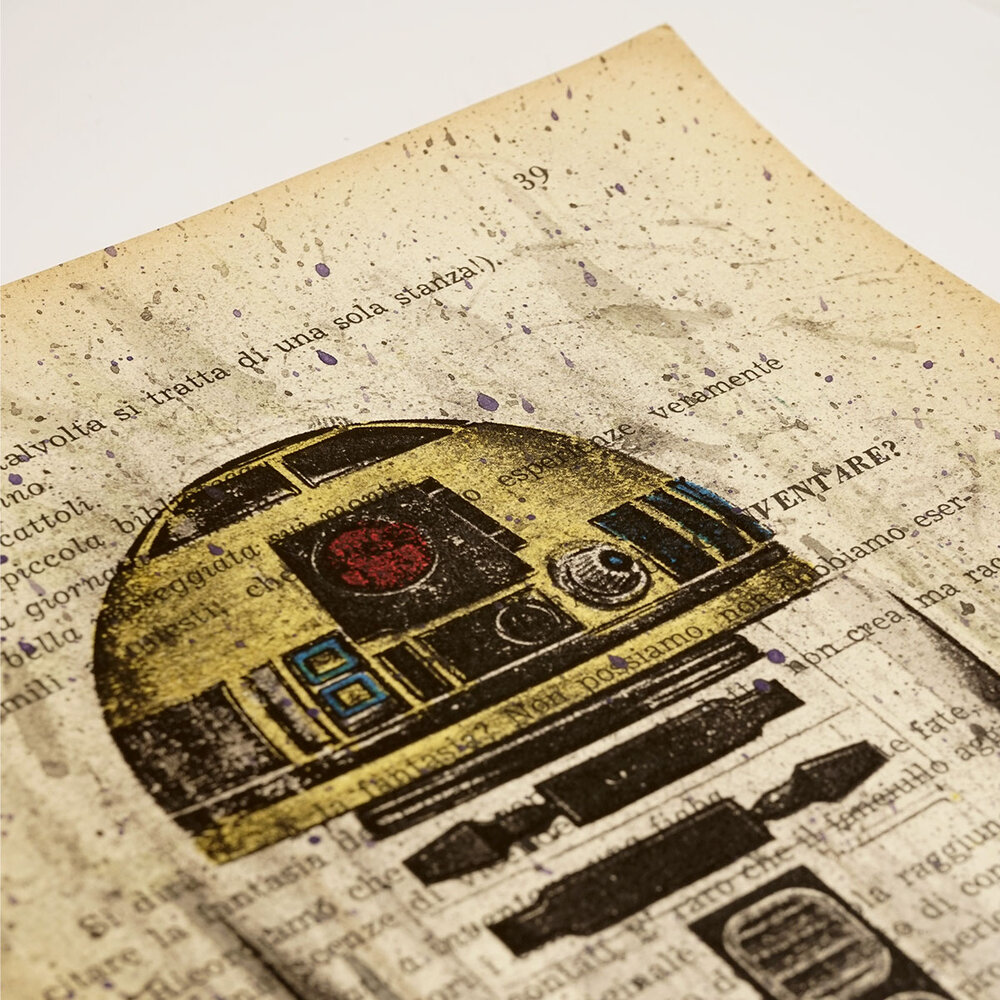



R2 D2 Filippo Imbrighi Artist




Christie O Doherty On Instagram You Re The Droid I M Looking For This Custom Piece Is Available O Star Wars Drawings Drawings For Boyfriend Star Wars Love
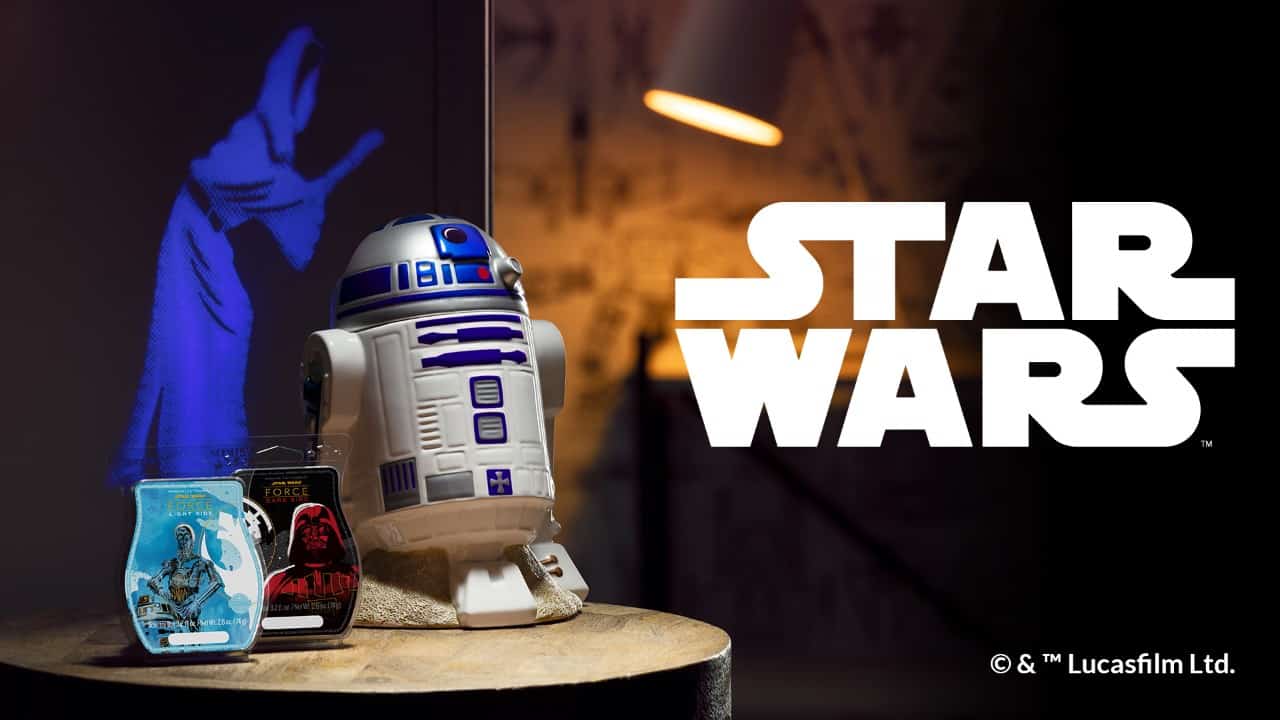



Star Wars Scentsy Collection R2 D2 Scentsy Warmer Shop Now Incandescent Scentsy Us
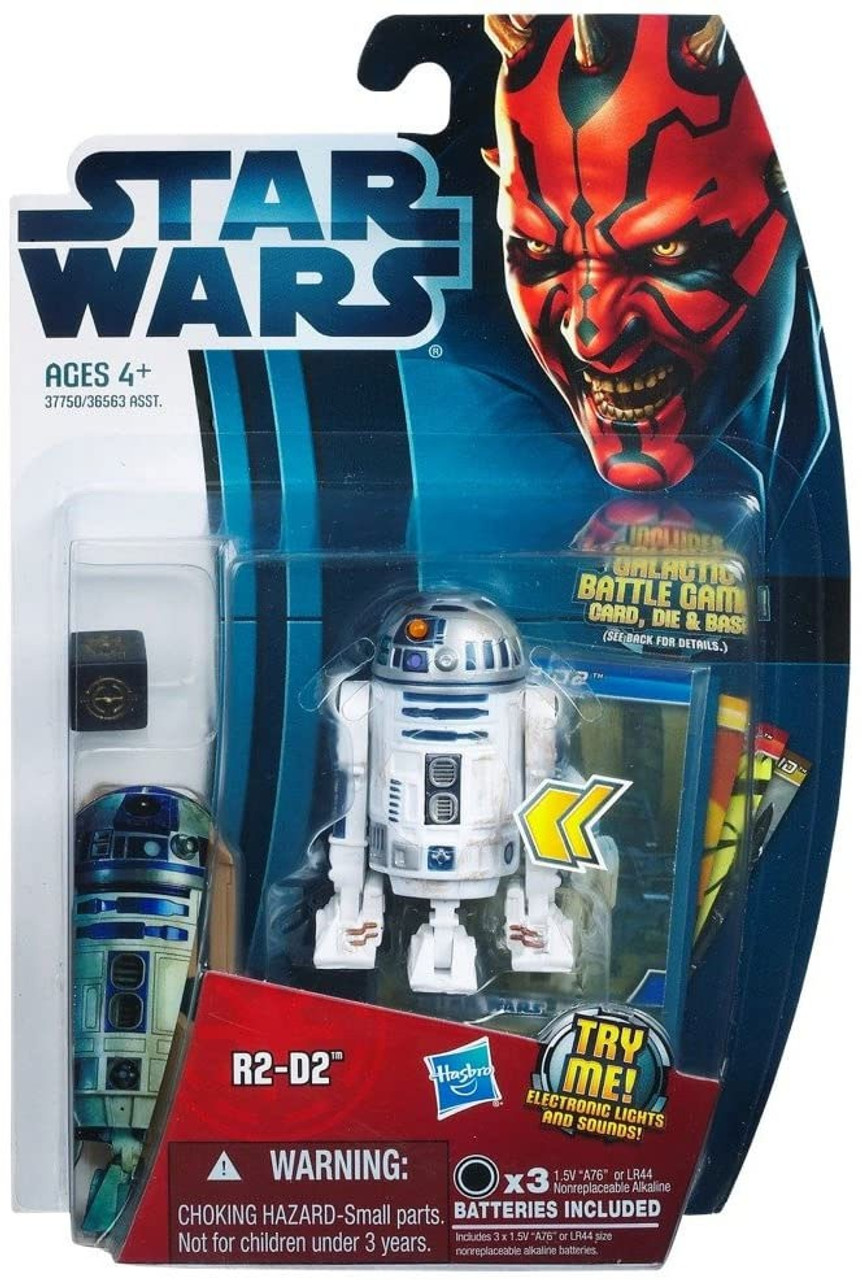



Star Wars Phantom Menace 12 Movie Heroes R2 D2 3 75 Action Figure 3 Hasbro Toys Toywiz




R2d2
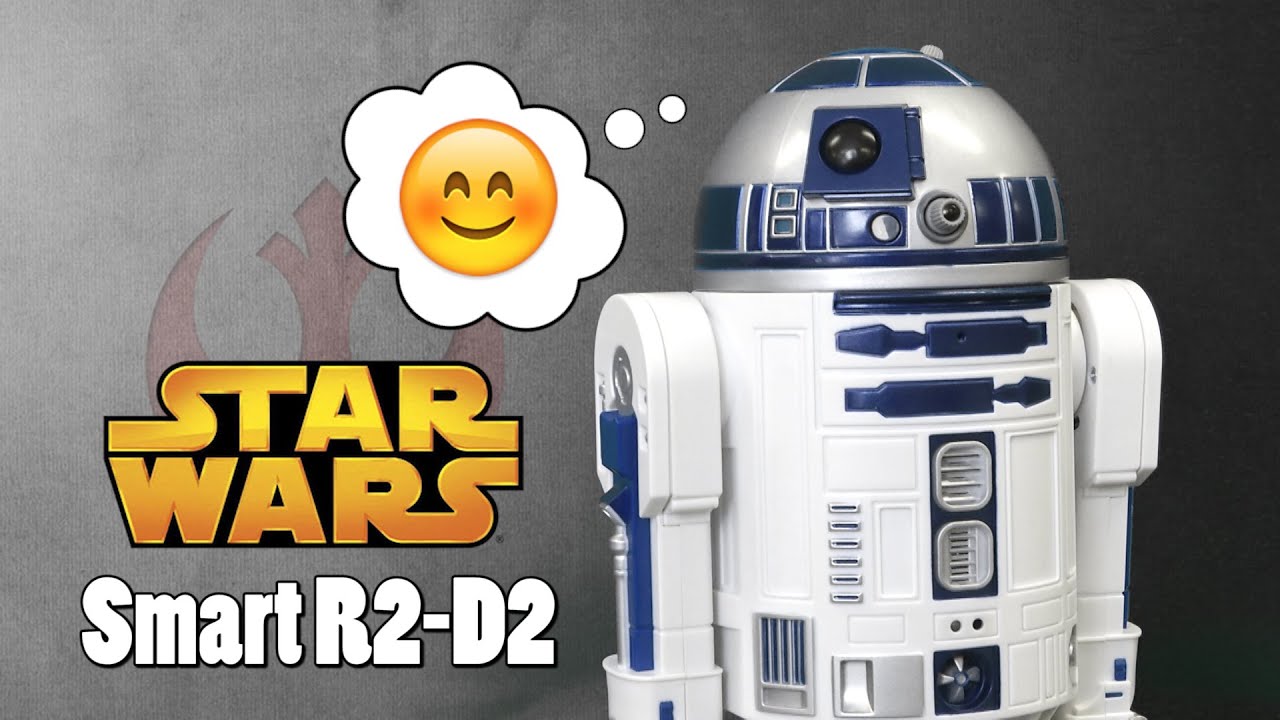



Star Wars Smart R2 D2 From Hasbro Youtube




The Blot Says R2 D2 Edition Star Wars Legion Stormtrooper Helmet 6 Vinyl Figure
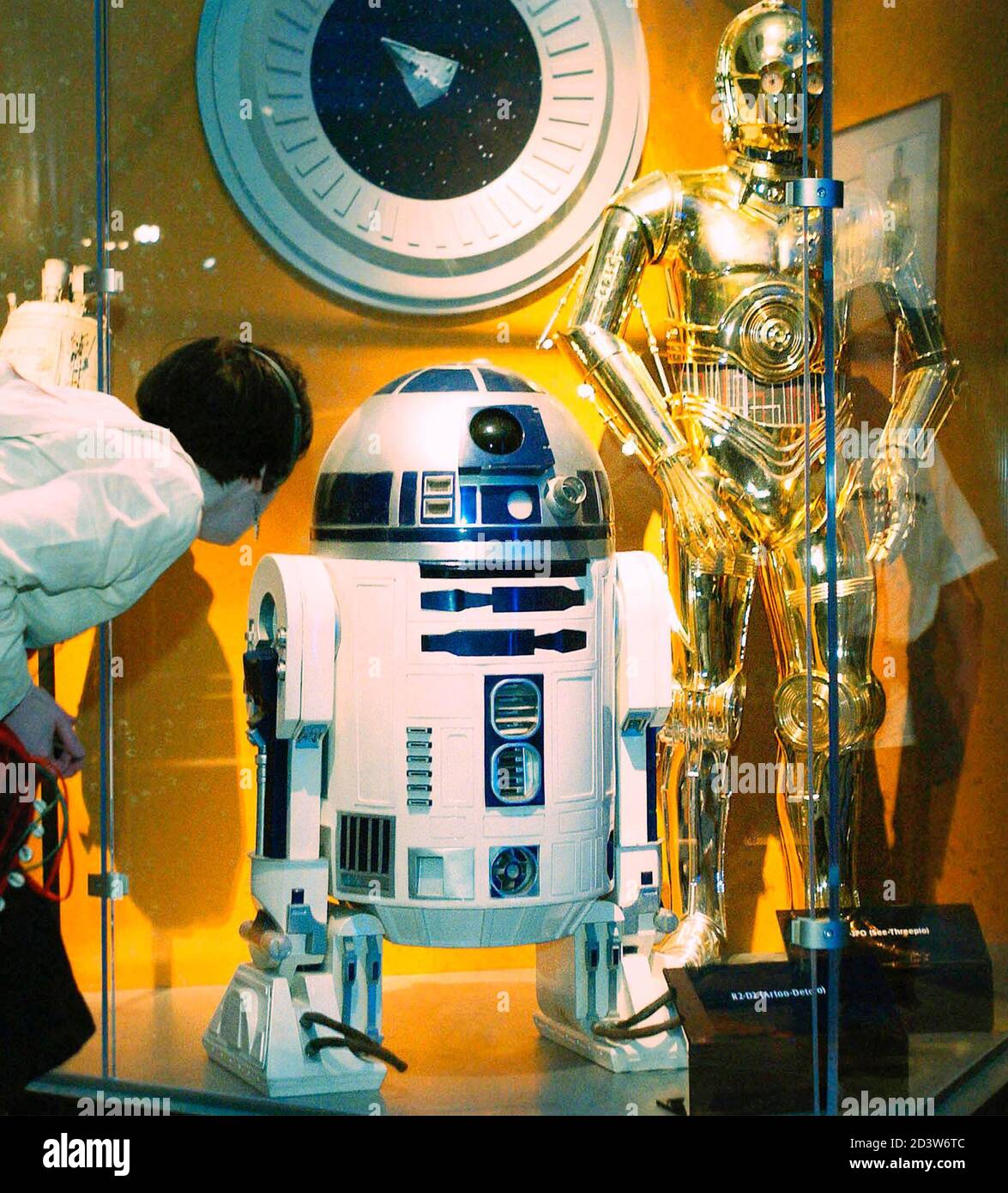



C3 Po High Resolution Stock Photography And Images Alamy




Star Wars The Force Awakens R2 D2 Hot Toys Machinegun
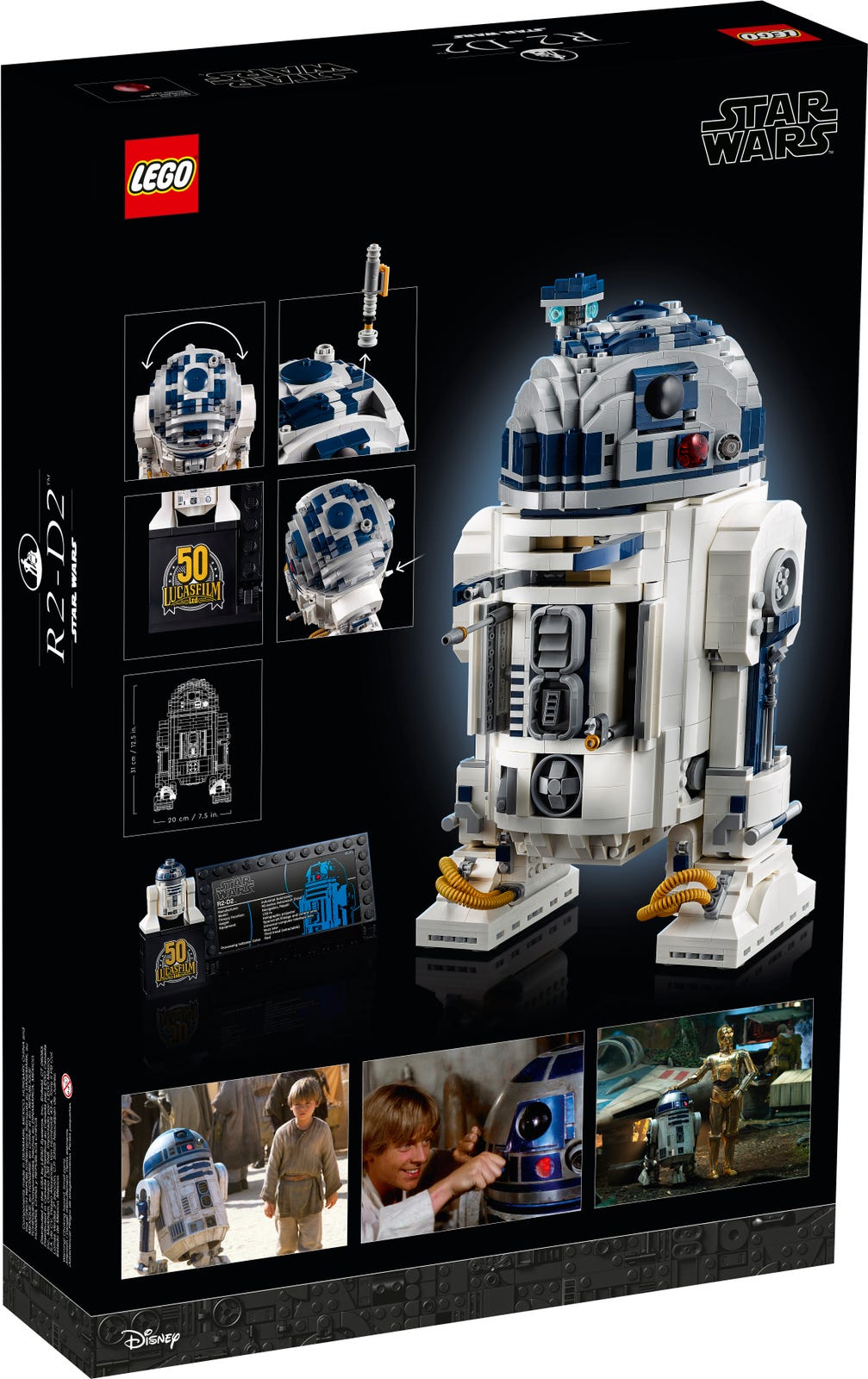



Lego R2 D2 Brickshop Lego En Duplo Specialist




Gc6f4fe R2 D2 Traditional Cache In Denmark Created By Isabellamolly
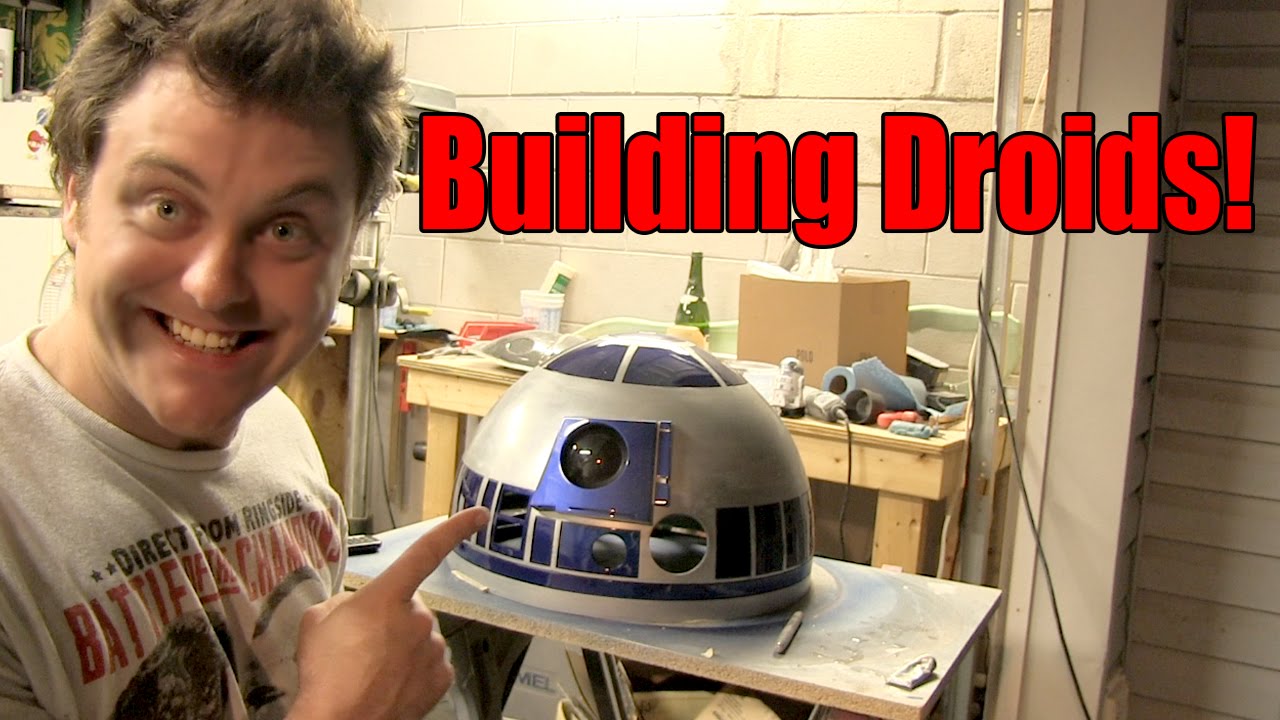



The Comprehensive Guide To Building A Realistic R2 D2 Replica Make




The Comprehensive Guide To Building A Realistic R2 D2 Replica Make




U R2 Cute Diy R2 D2 Costume May The Fourth Be With You Party
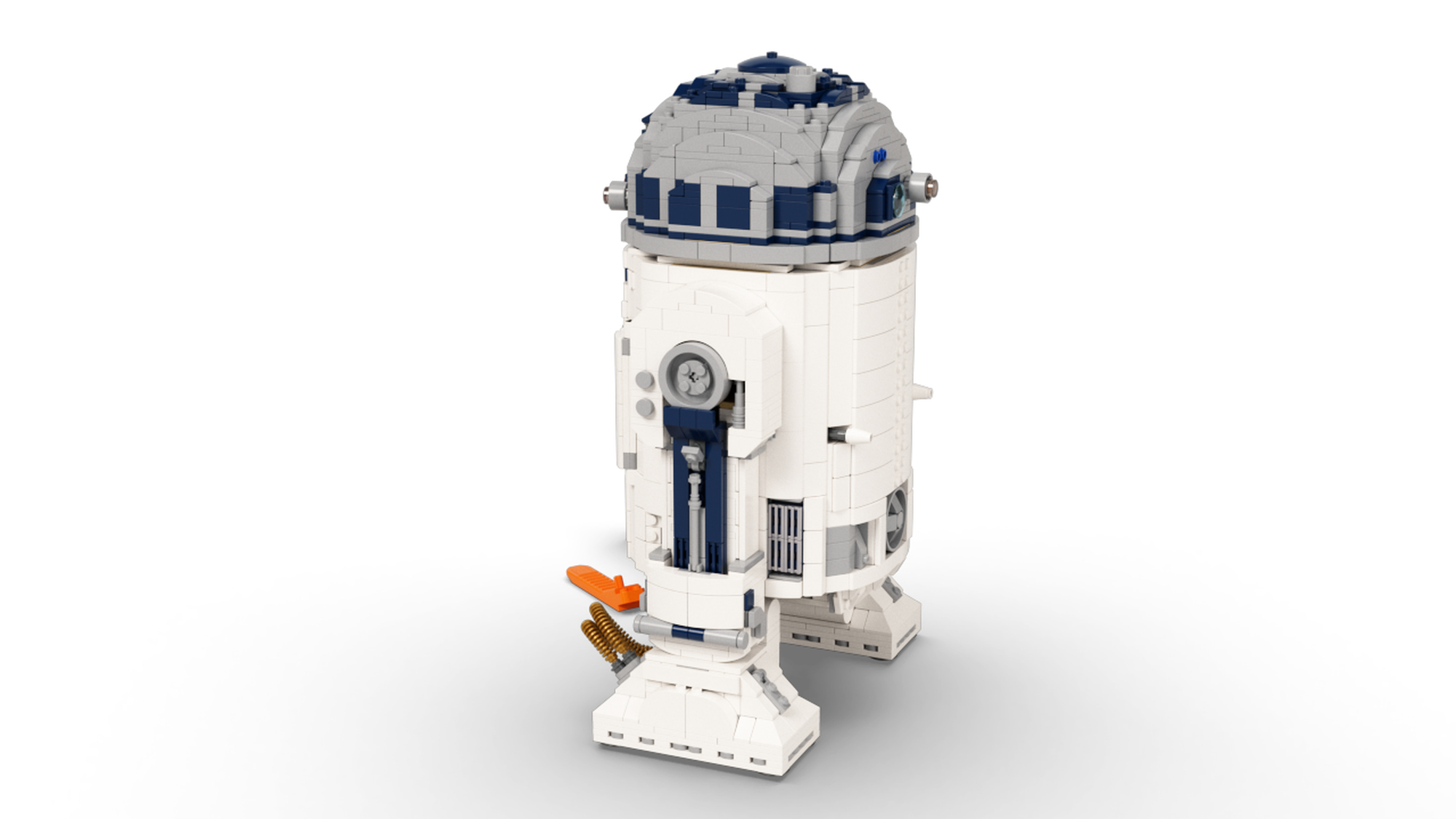



Lego R2 D2 Brickshop Lego En Duplo Specialist



R2 D2 Filippo Imbrighi Artist
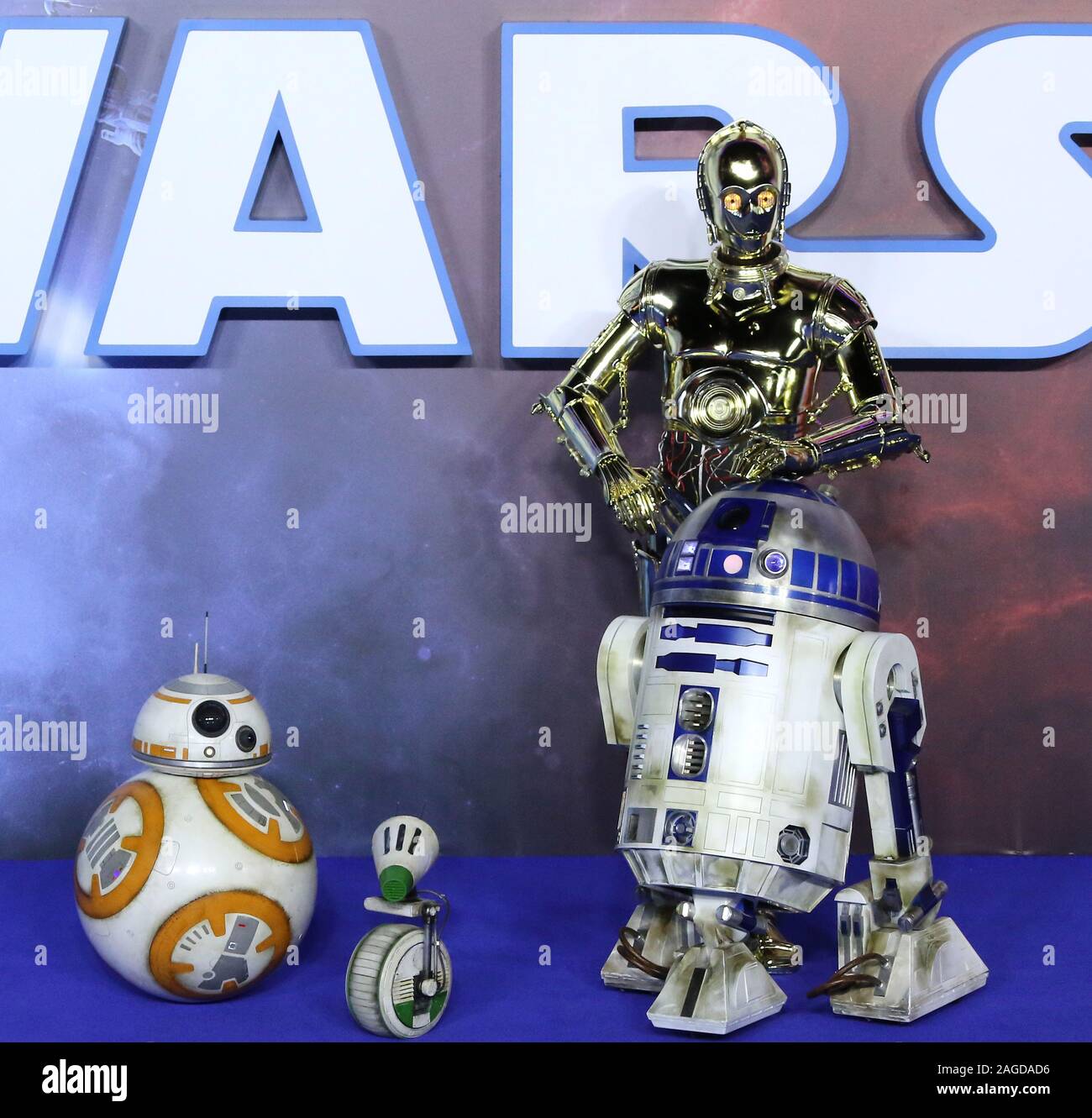



8 And D O High Resolution Stock Photography And Images Alamy
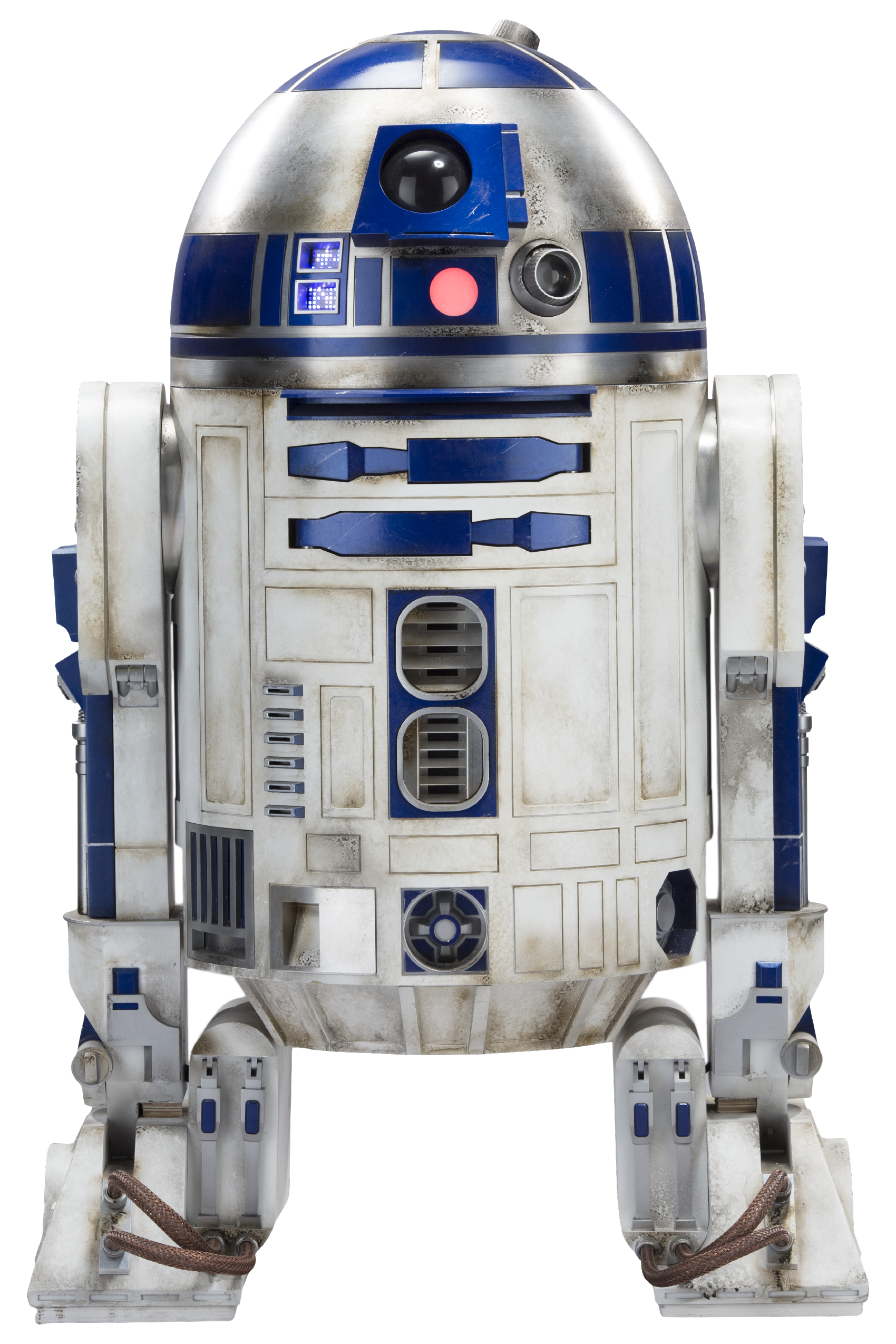



R2 D2 Wookieepedia Fandom




Amazon Com Star Wars Episode 1 R2 D2 Carryall Playset With Exclusive Destroyer Droid Figure Toys Games




Medicom Mafex Star Wars R2 D2 And C 3po Fwoosh
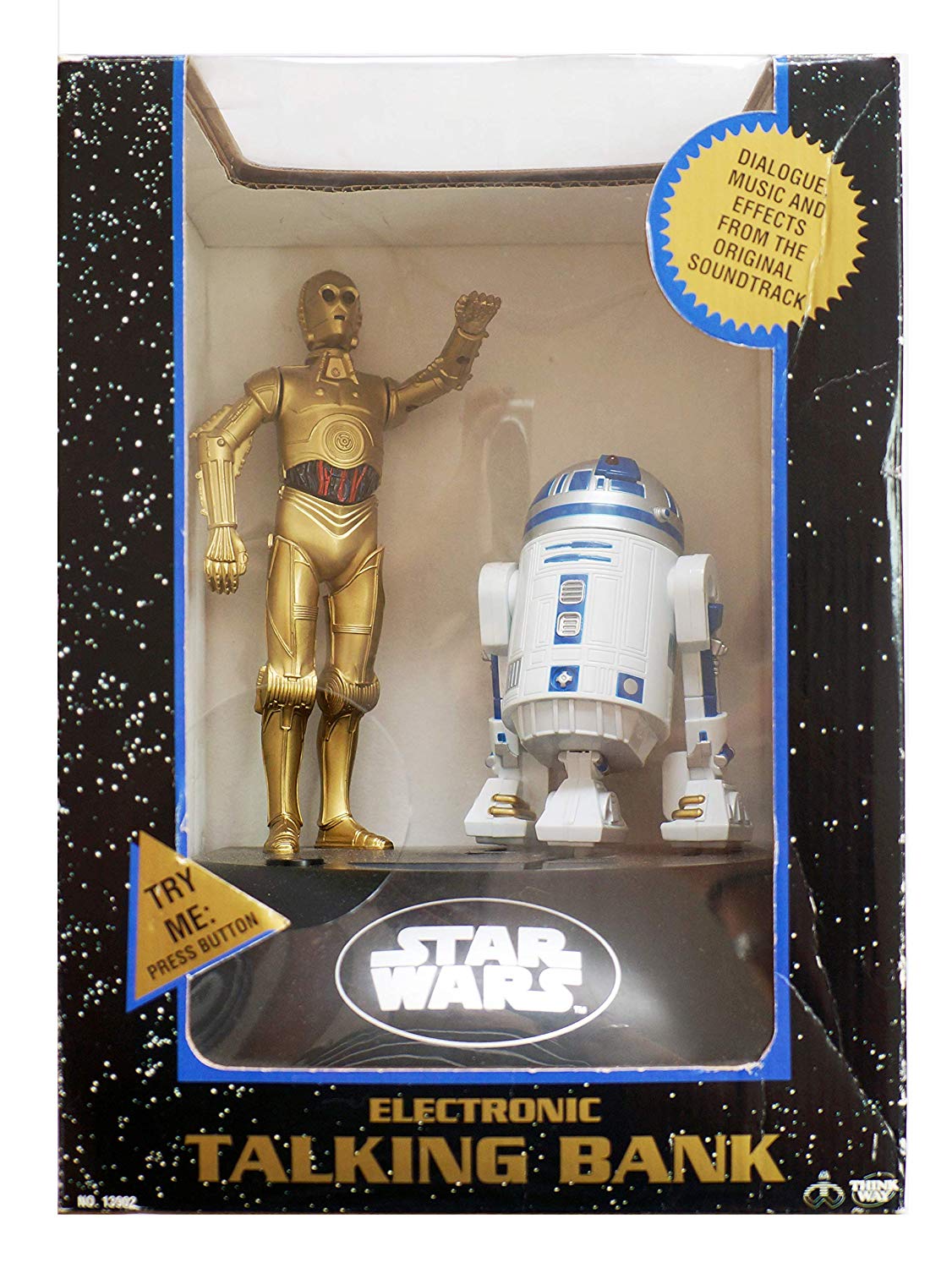



Electronic Talking Bank C 3po R2 D2 Star Wars The Empire Strikes Back Thinkway Vintage Collection Rare Vintage 1995 Now And Then Collectibles
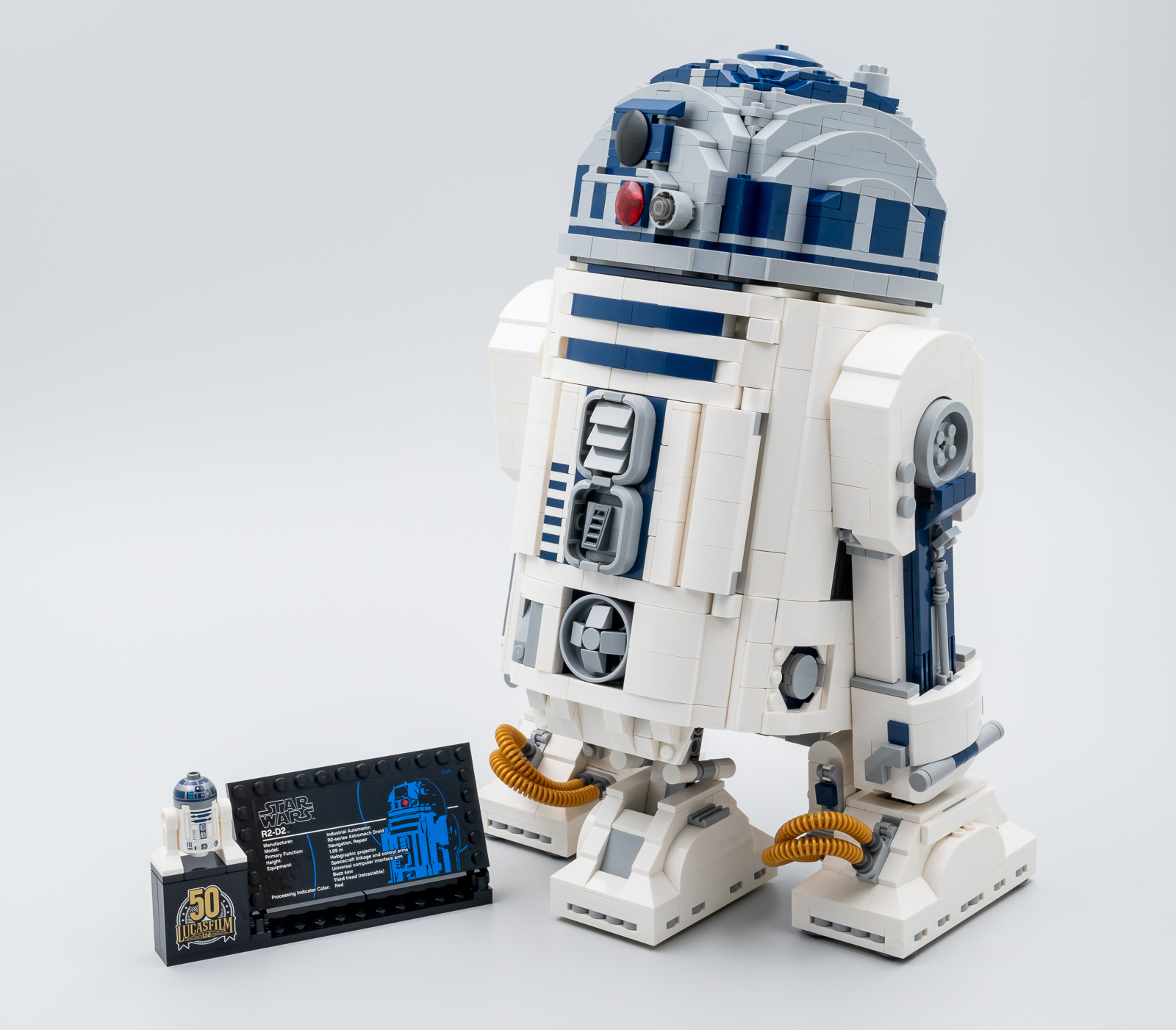



Review Lego Star Wars R2 D2 Hoth Bricks




Judith Leiber Couture R2d2 Crystal Clutch Bag Neiman Marcus
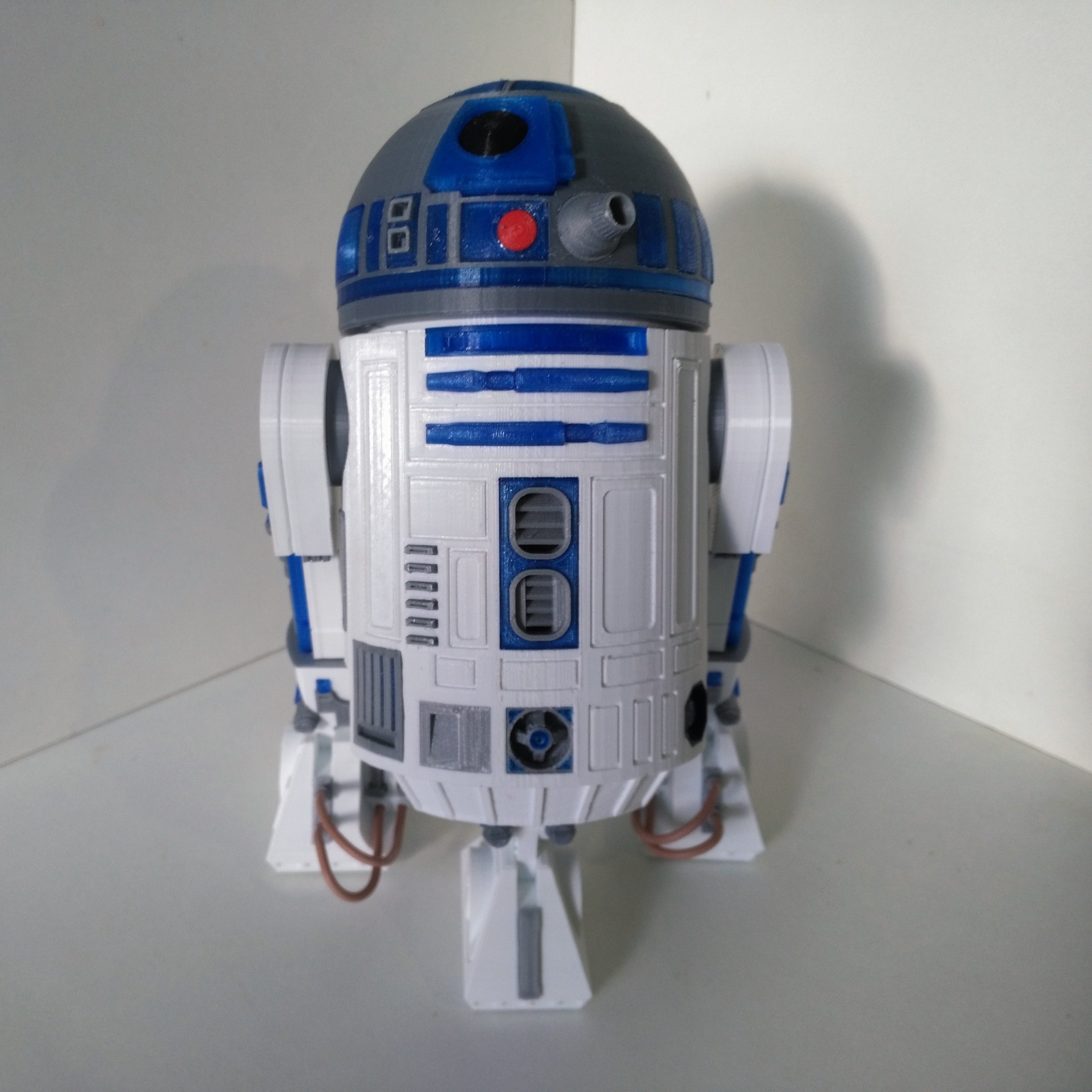



3d Printed Star Wars R2d2 Detailed Printable Rotating Openable Head By Mochiczuki Pinshape



6ooo1 Wireless Remote Control Toy Tx User Manual Btsw001 Target Manual R2 D2 Outlined Virztex
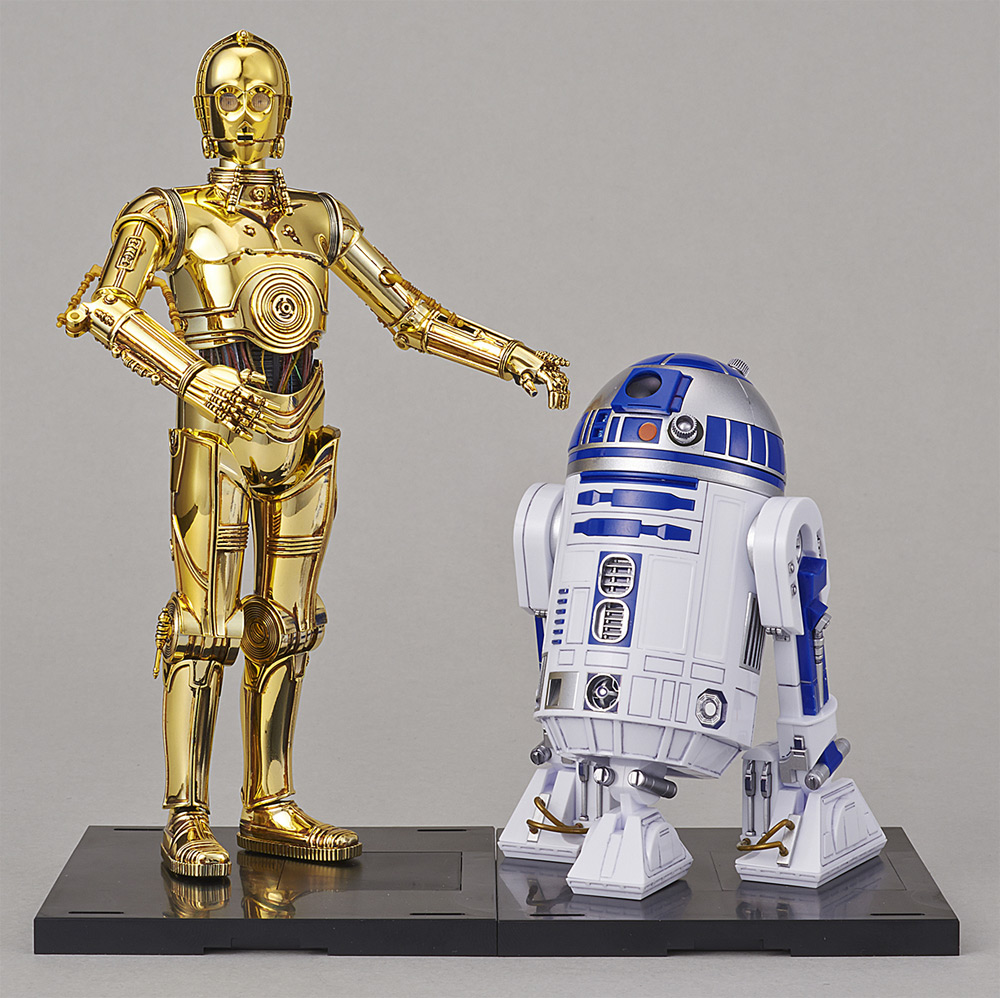



Star Wars The Last Jedi C 3po And R2 D2 1 12 Scale Model Kit By Bandai Star Wars The Last Jedi C 3po And R2 D2 1 12 Scale Model Kit By Bandai 26wba63 35 99




Medicom Mafex Star Wars R2 D2 And C 3po Fwoosh




R2 D2 Skilled Starship Mechanic Star Wars Buddies Episodes I Thru Viii Plus Others The Kenner Vintage Collection Rare Vintage 1997 Now And Then Collectibles
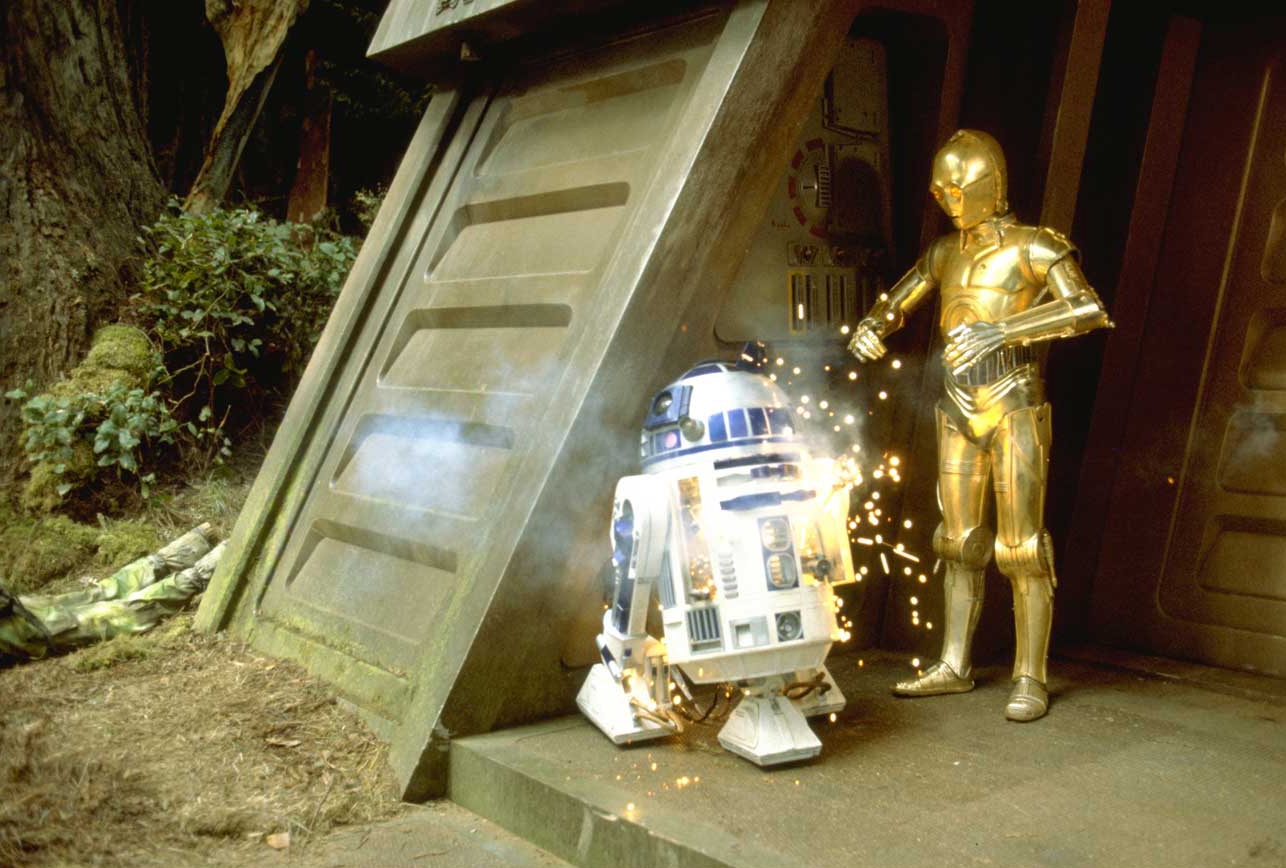



R2 D2 Wookieepedia Fandom
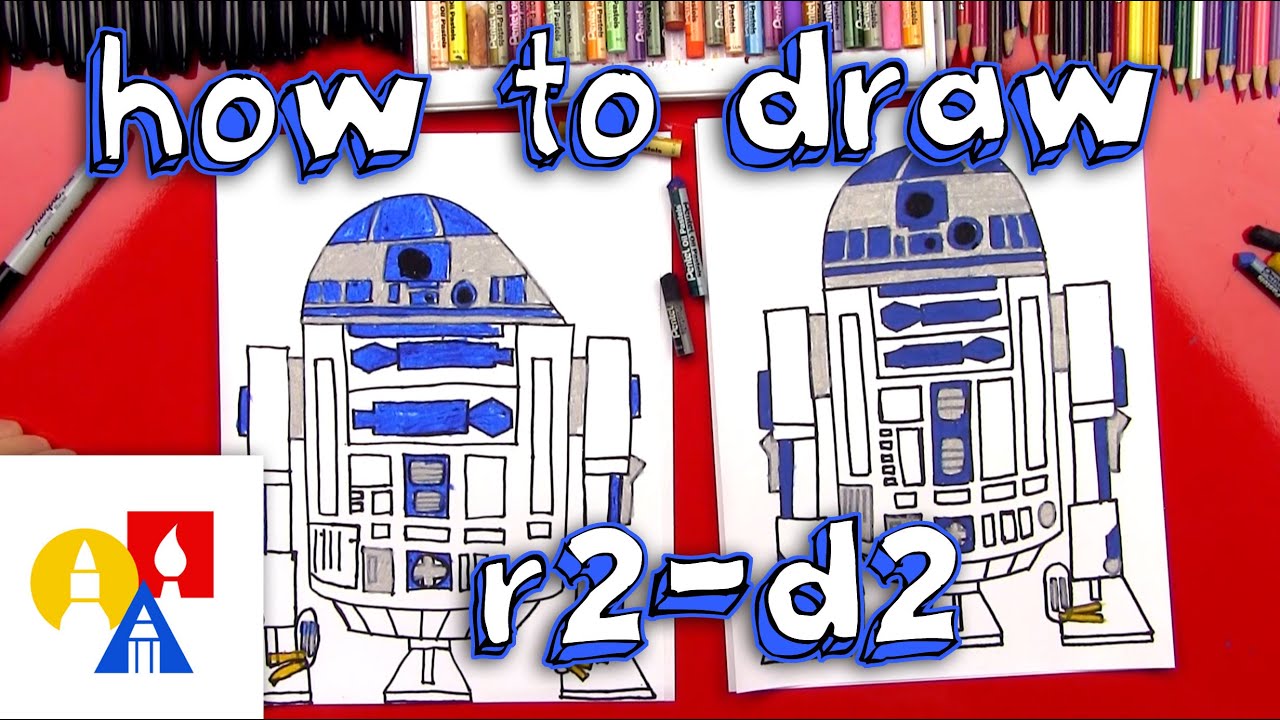



How To Draw R2 D2 Youtube
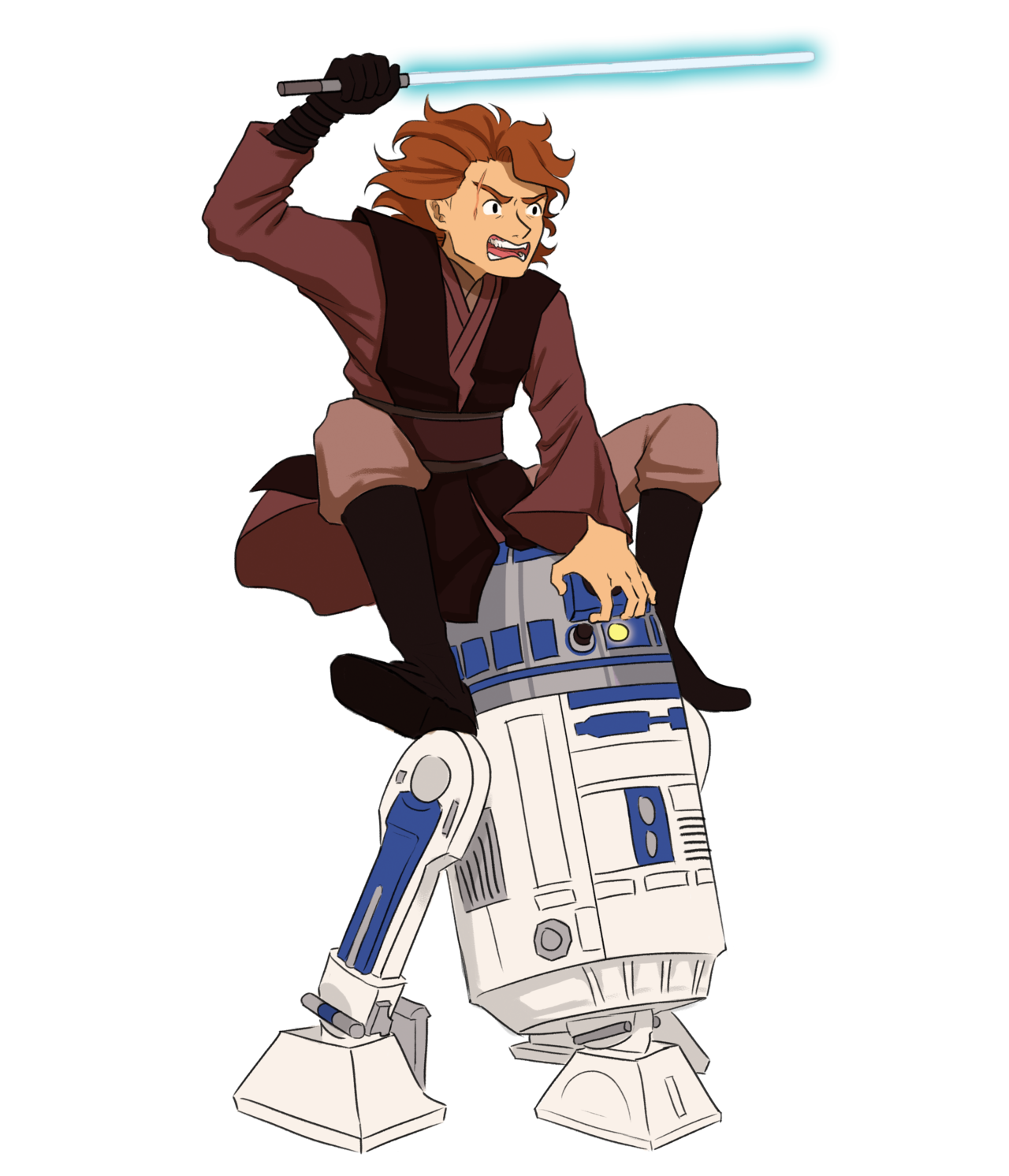



Padawanlost Sidlaux Draw Anakin Skywalker Riding R2d2




Star Wars Blueprints 01 R2 D2 Side Elevation In 21 Star Wars Pictures Star Wars Droids Star Wars Spaceships
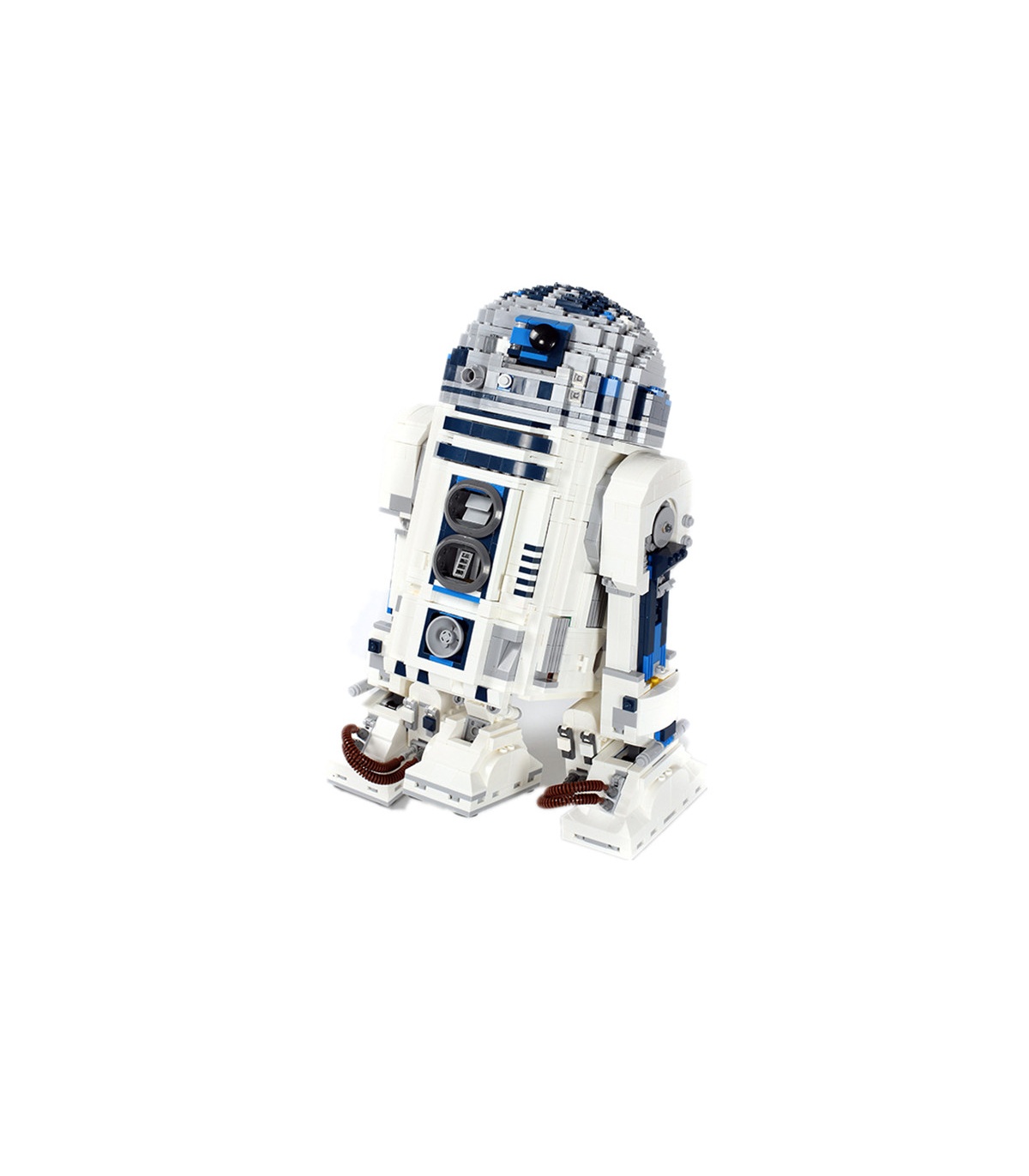



Custom Star Wars R2 D2 Compatible Building Bricks Toy Set 2127 Pieces Buildingtoystore Com
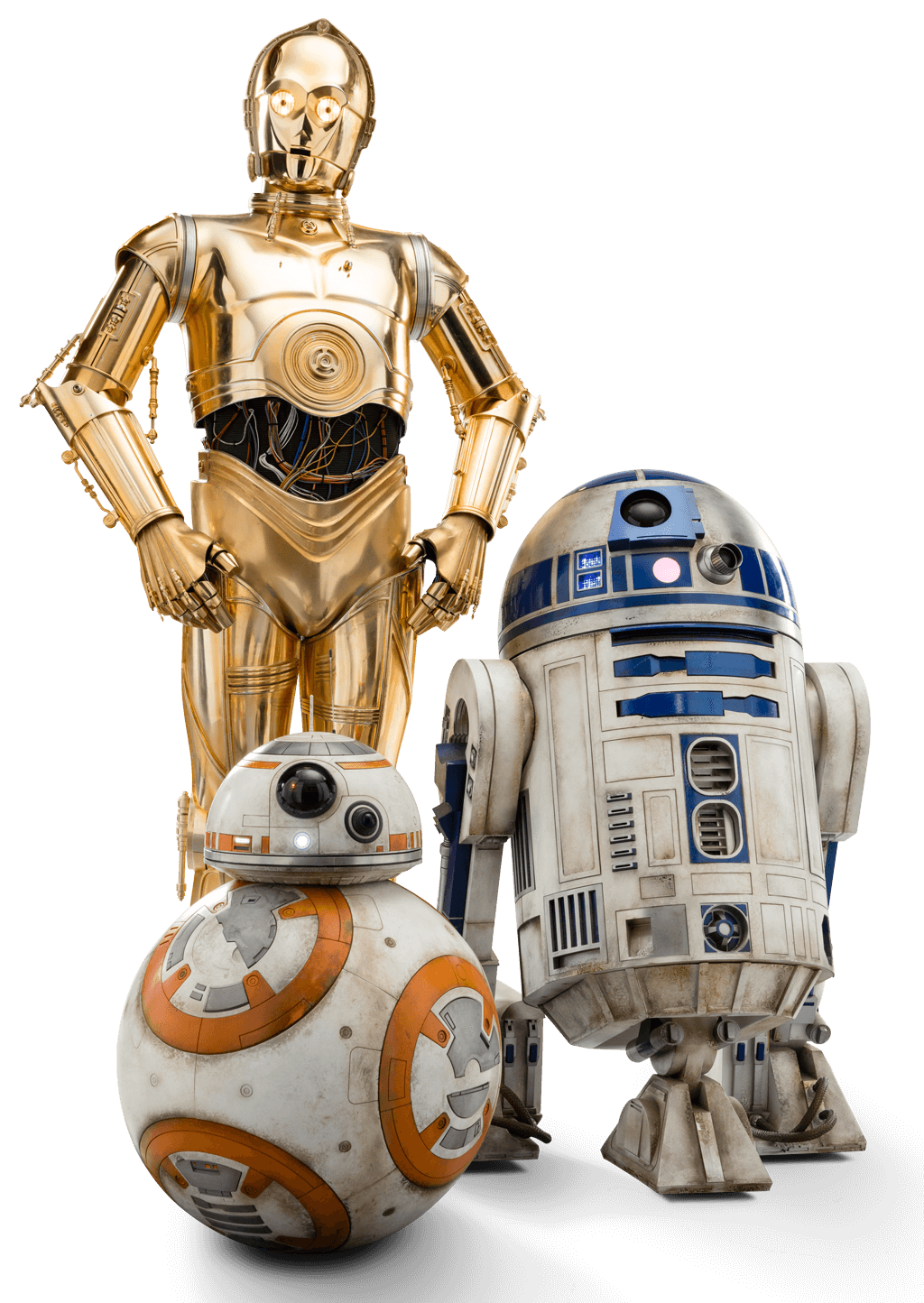



Droid Wookieepedia Fandom
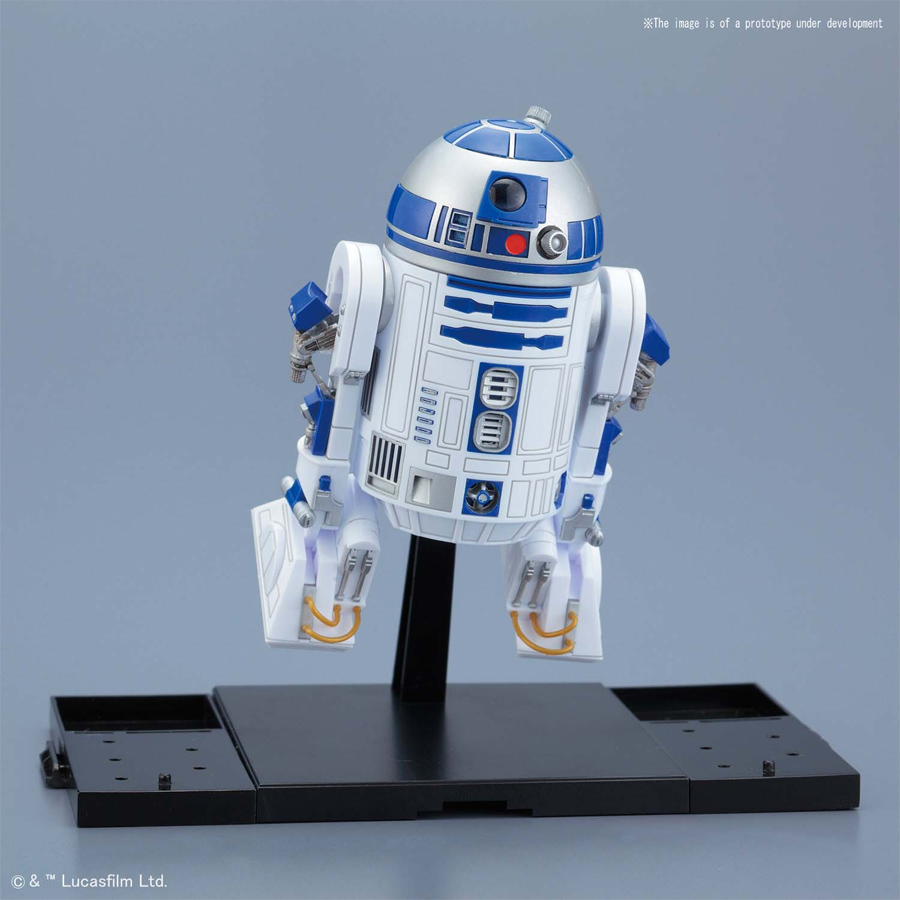



Star Wars Droid Collection R2 D2 Rocket Booster Version 1 12 Scale Model Kit Star Wars Droid Collection R2 D2 Rocket Booster Version 1 12 Scale Model Kit 26wba71 24 99 Monsters In Motion Movie



Tales To Astonish Star Wars




Medicom Mafex Star Wars R2 D2 And C 3po Fwoosh
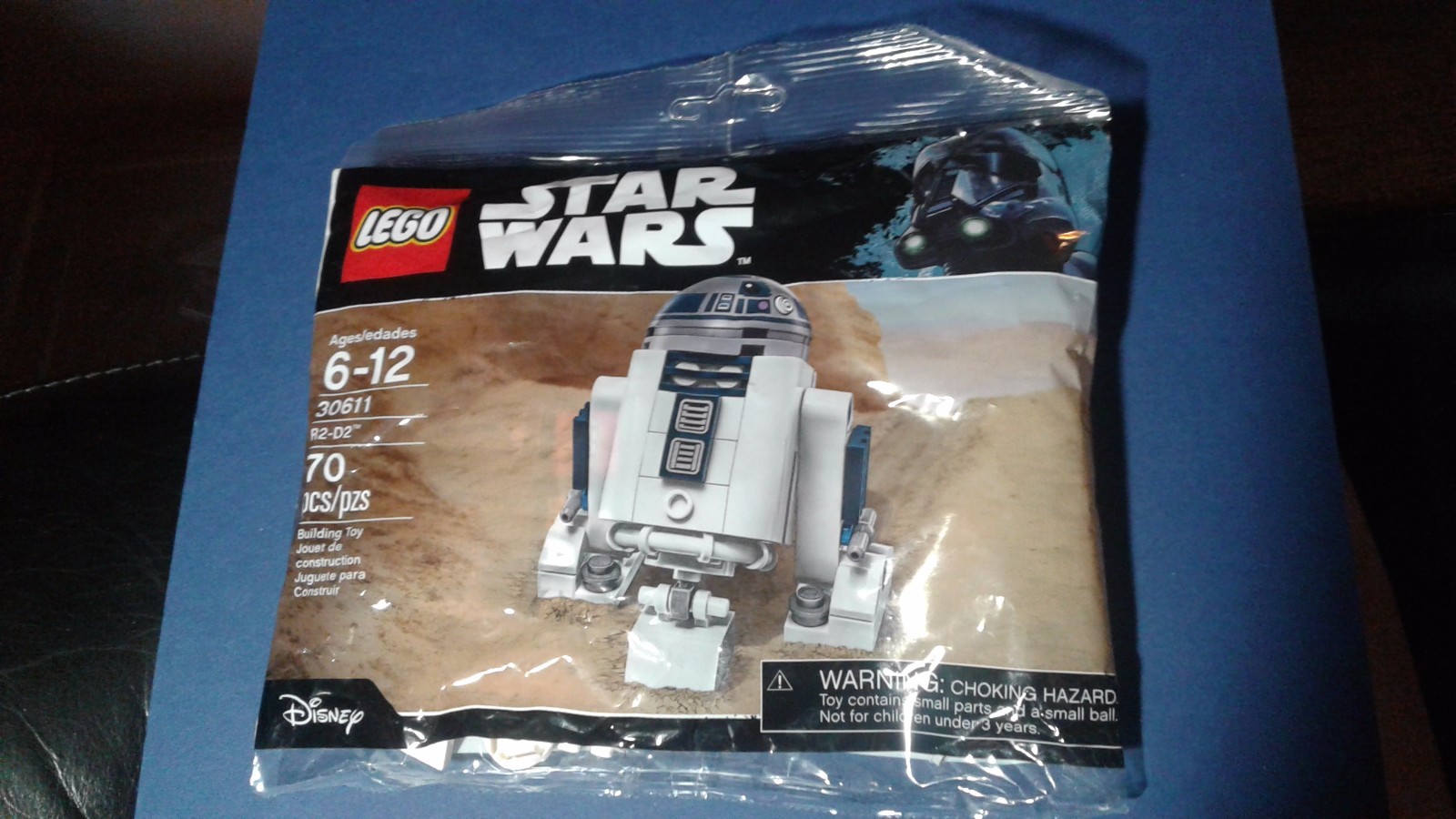



Lego Star Wars R2 D2 17 70pcs Polybag Retired For Sale Online Ebay




Star Wars R2 D2 Premium Format
0 件のコメント:
コメントを投稿